Evidence
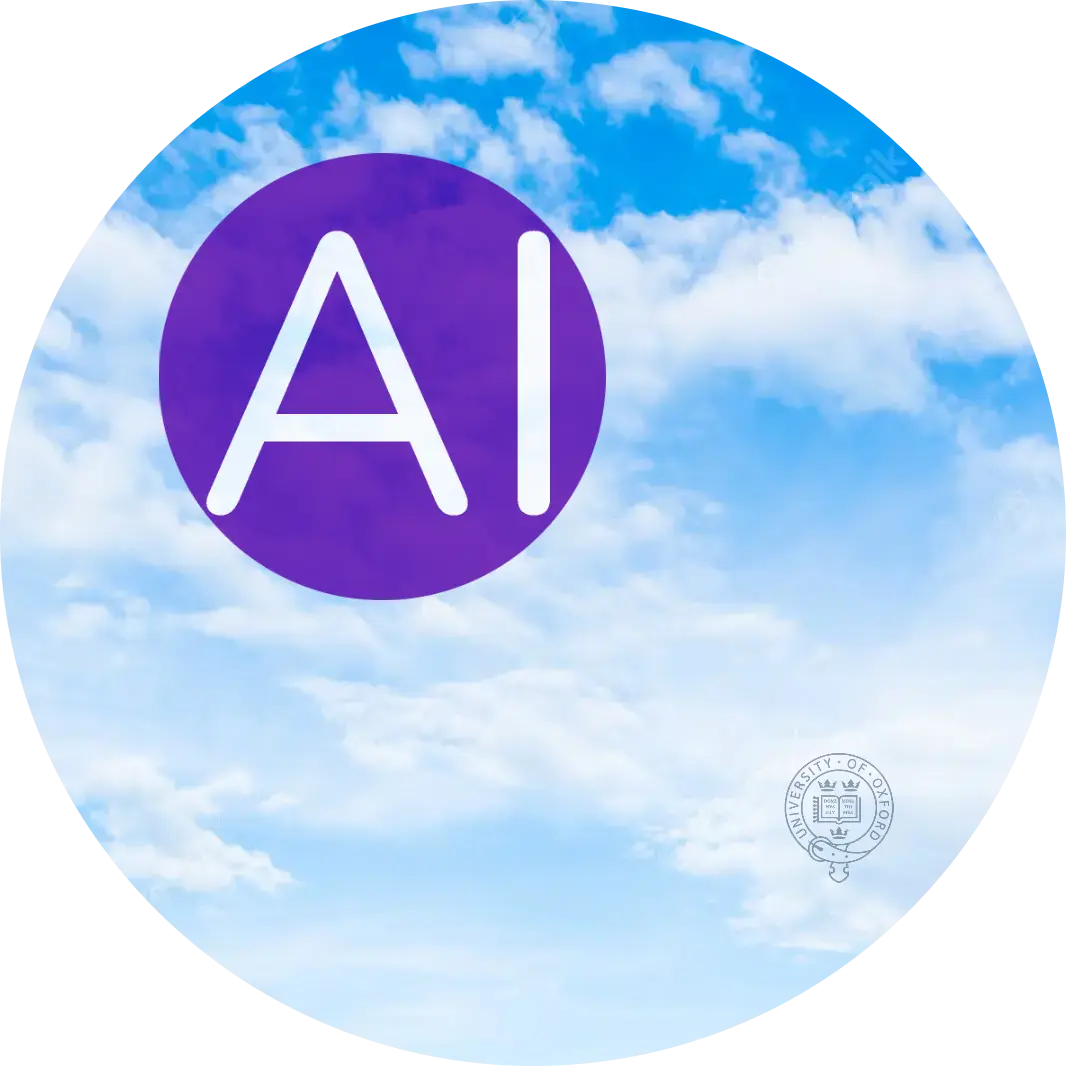
medRxiv [Preprint]. 2024 Apr 24:2024.04.23.24306225. doi: 10.1101/2024.04.23.24306225.
ABSTRACT
OBJECTIVE: To assess the accuracy of a large language model (LLM) in measuring clinician adherence to practice guidelines for monitoring side effects after prescribing medications for children with attention-deficit/hyperactivity disorder (ADHD).
METHODS: Retrospective population-based cohort study of electronic health records. Cohort included children aged 6-11 years with ADHD diagnosis and ≥2 ADHD medication encounters (stimulants or non-stimulants prescribed) between 2015-2022 in a community-based primary healthcare network (n=1247). To identify documentation of side effects inquiry, we trained, tested, and deployed an open-source LLM (LLaMA) on all clinical notes from ADHD-related encounters (ADHD diagnosis or ADHD medication prescription), including in-clinic/telehealth and telephone encounters (n=15,593 notes). Model performance was assessed using holdout and deployment test sets, compared to manual chart review.
RESULTS: The LLaMA model achieved excellent performance in classifying notes that contain side effects inquiry (sensitivity= 87.2%, specificity=86.3/90.3%, area under curve (AUC)=0.93/0.92 on holdout/deployment test sets). Analyses revealed no model bias in relation to patient age, sex, or insurance. Mean age (SD) at first prescription was 8.8 (1.6) years; patient characteristics were similar across patients with and without documented side effects inquiry. Rates of documented side effects inquiry were lower in telephone encounters than in-clinic/telehealth encounters (51.9% vs. 73.0%, p<0.01). Side effects inquiry was documented in 61% of encounters following stimulant prescriptions and 48% of encounters following non-stimulant prescriptions (p<0.01).
CONCLUSIONS: Deploying an LLM on a variable set of clinical notes, including telephone notes, offered scalable measurement of quality-of-care and uncovered opportunities to improve psychopharmacological medication management in primary care.
PMID:38712037 | PMC:PMC11071552 | DOI:10.1101/2024.04.23.24306225



Estimated reading time: 5 minute(s)
Latest: Psychiatryai.com #RAISR4D

Cool Evidence: Engaging Young People and Students in Real-World Evidence ☀️

Real-Time Evidence Search [Psychiatry]

AI Research [Andisearch.com]

Leveraging a Large Language Model to Assess Quality-of-Care: Monitoring ADHD Medication Side Effects
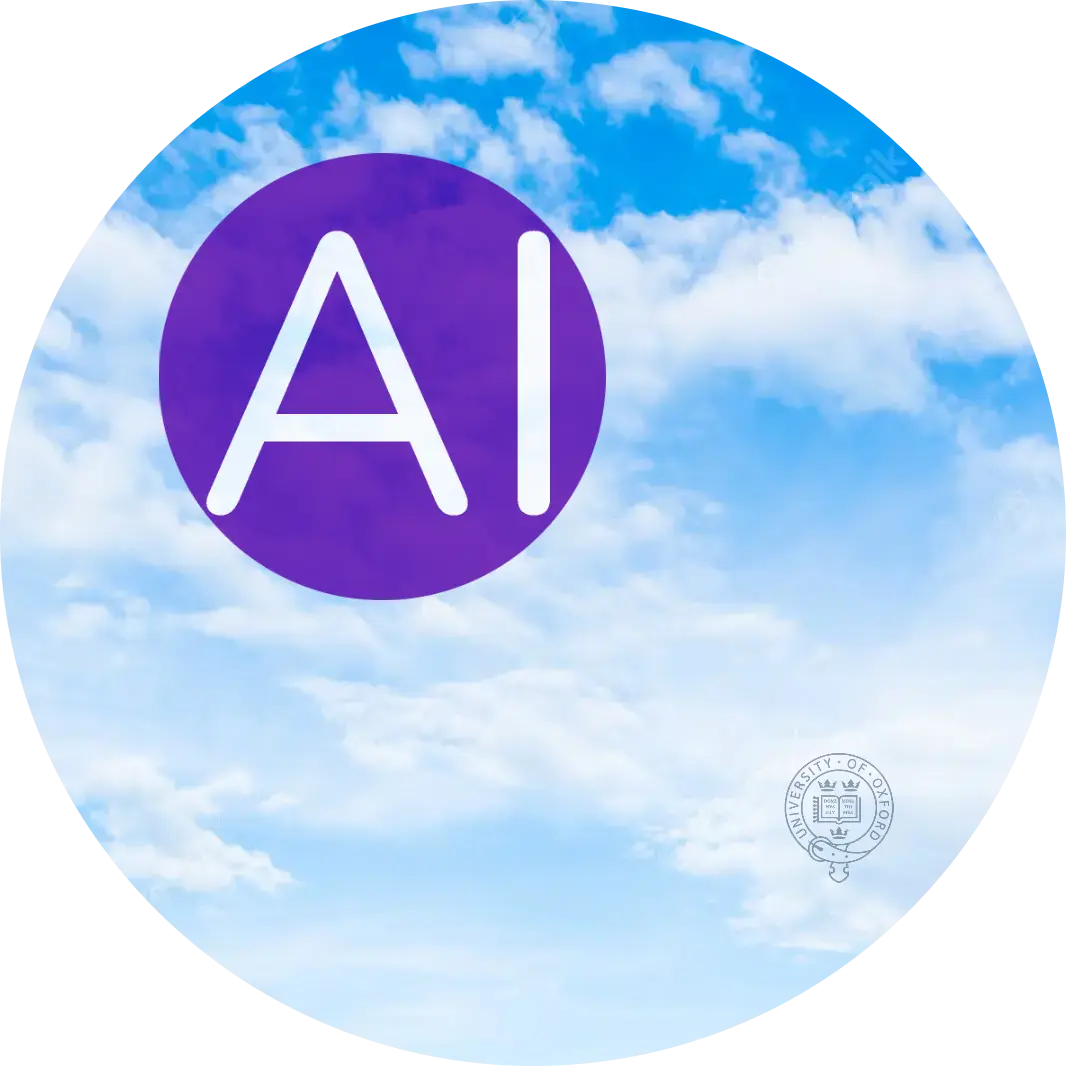
🌐 90 Days
Evidence Blueprint
Leveraging a Large Language Model to Assess Quality-of-Care: Monitoring ADHD Medication Side Effects
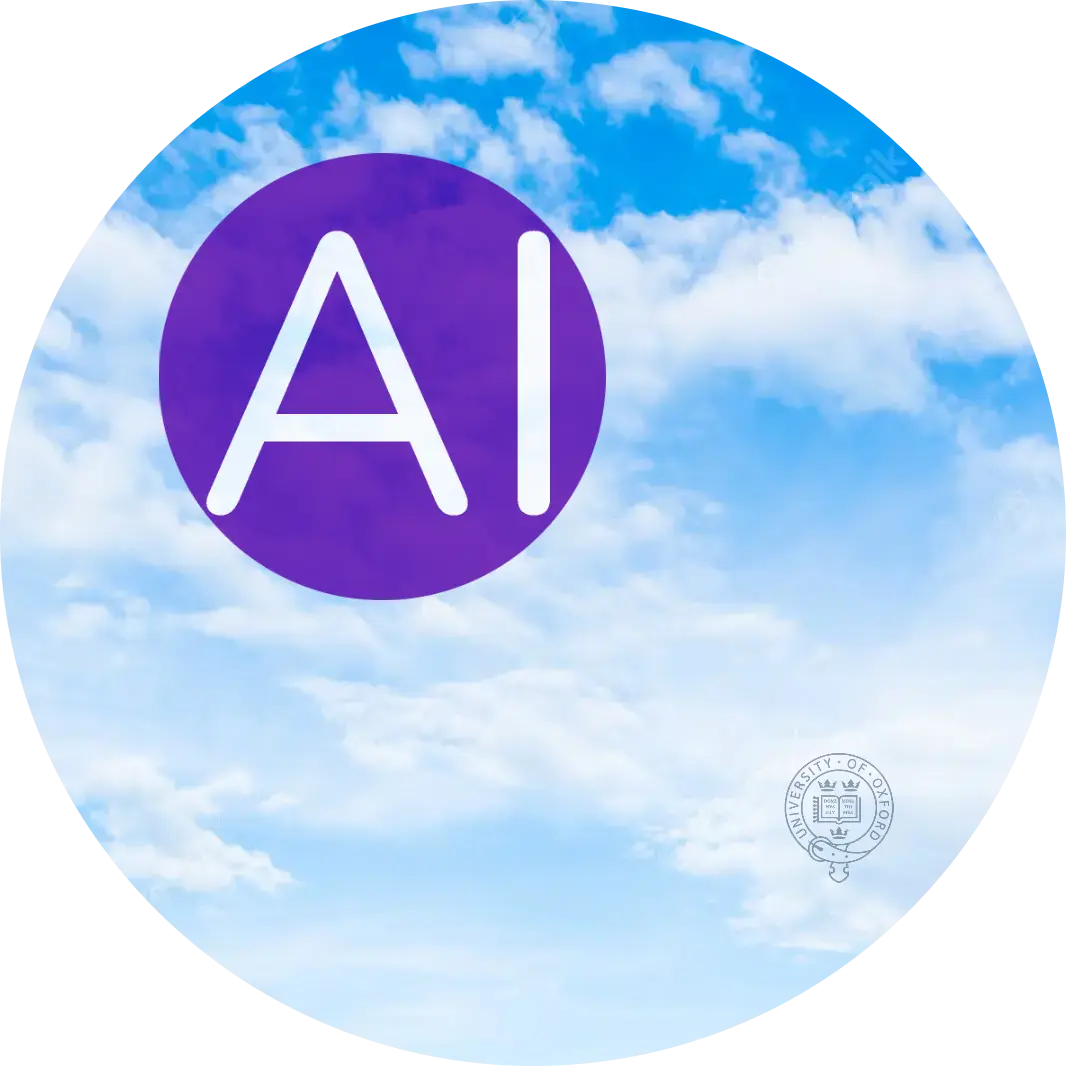
☊ AI-Driven Related Evidence Nodes
(recent articles with at least 5 words in title)
More Evidence
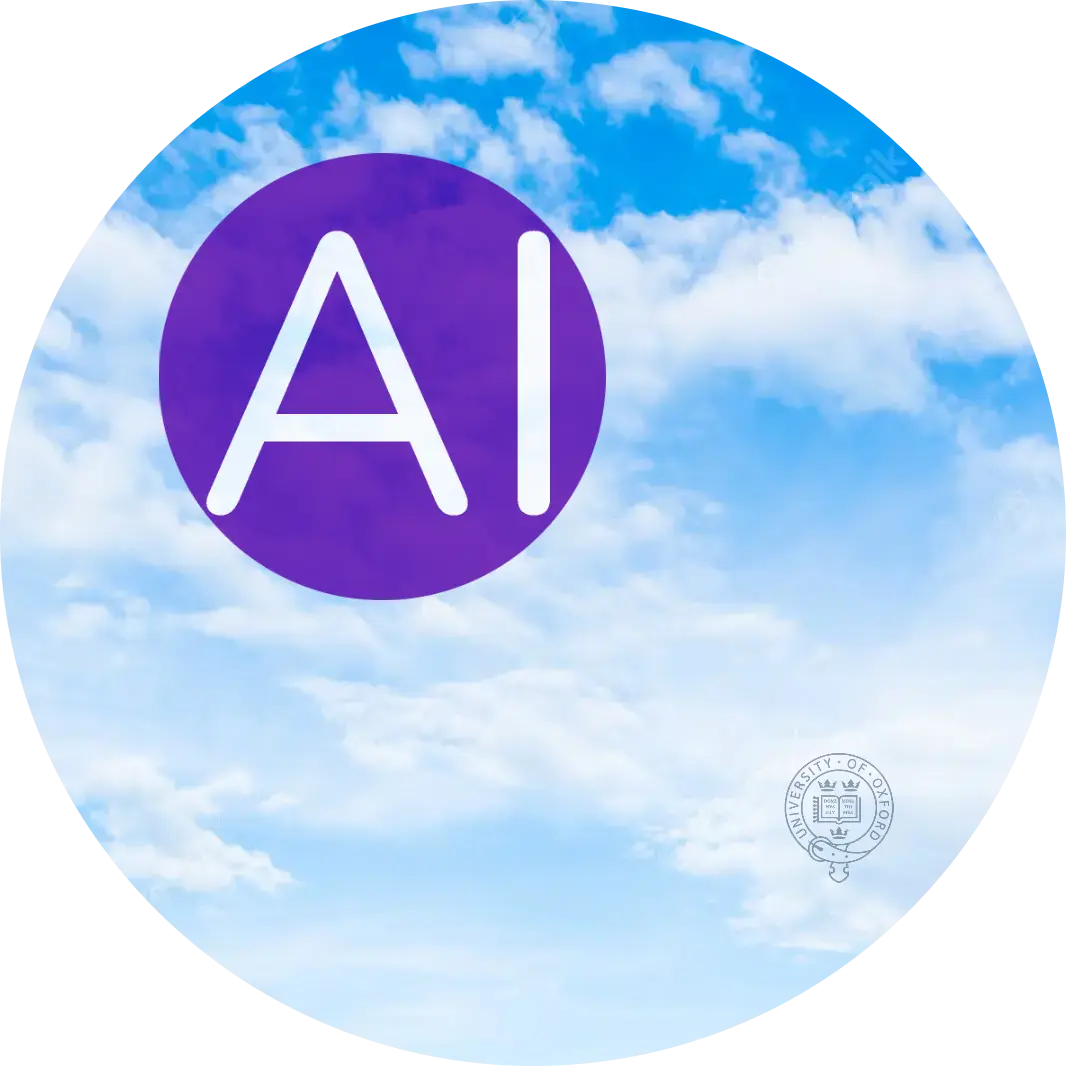