Evidence
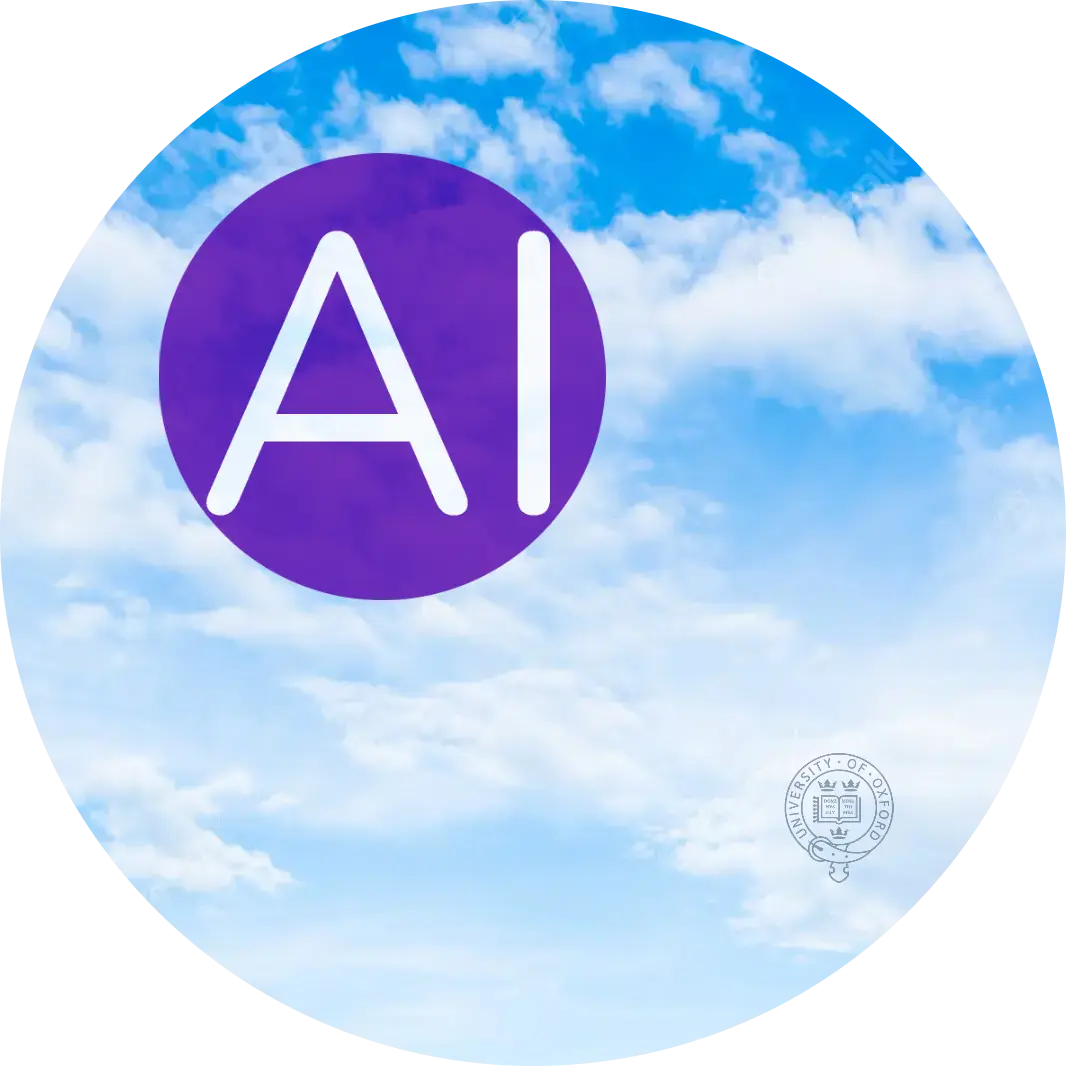
J Affect Disord. 2024 May 26:S0165-0327(24)00868-1. doi: 10.1016/j.jad.2024.05.125. Online ahead of print.
ABSTRACT
BACKGROUND: Functional connectivity has garnered interest as a potential biomarker of psychiatric disorders including borderline personality disorder (BPD). However, small sample sizes and lack of within-study replications have led to divergent findings with no clear spatial foci.
AIMS: Evaluate discriminative performance and generalizability of functional connectivity markers for BPD.
METHOD: Whole-brain fMRI resting state functional connectivity in matched subsamples of 116 BPD and 72 control individuals defined by three grouping strategies. We predicted BPD status using classifiers with repeated cross-validation based on multiscale functional connectivity within and between regions of interest (ROIs) covering the whole brain-global ROI-based network, seed-based ROI-connectivity, functional consistency, and voxel-to-voxel connectivity-and evaluated the generalizability of the classification in the left-out portion of non-matched data.
RESULTS: Full-brain connectivity allowed classification (~70 %) of BPD patients vs. controls in matched inner cross-validation. The classification remained significant when applied to unmatched out-of-sample data (~61-70 %). Highest seed-based accuracies were in a similar range to global accuracies (~70-75 %), but spatially more specific. The most discriminative seed regions included midline, temporal and somatomotor regions. Univariate connectivity values were not predictive of BPD after multiple comparison corrections, but weak local effects coincided with the most discriminative seed-ROIs. Highest accuracies were achieved with a full clinical interview while self-report results remained at chance level.
LIMITATIONS: The accuracies vary considerably between random sub-samples of the population, global signal and covariates limiting the practical applicability.
CONCLUSIONS: Spatially distributed functional connectivity patterns are moderately predictive of BPD despite heterogeneity of the patient population.
PMID:38806064 | DOI:10.1016/j.jad.2024.05.125



Estimated reading time: 5 minute(s)
Latest: Psychiatryai.com #RAISR4D Evidence

Cool Evidence: Engaging Young People and Students in Real-World Evidence

Real-Time Evidence Search [Psychiatry]

AI Research

A machine-learning approach for differentiating borderline personality disorder from community participants with brain-wide functional connectivity
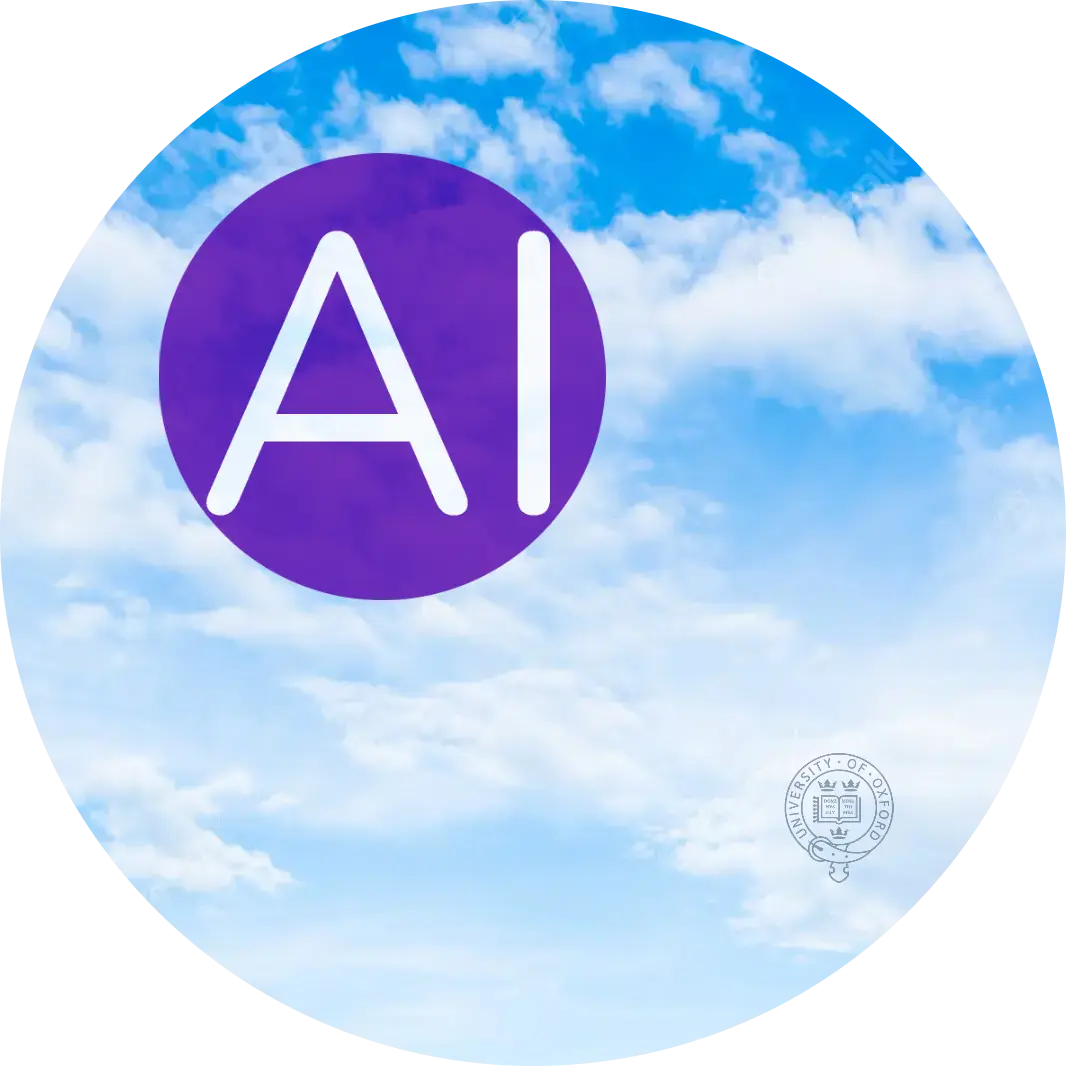
🌐 90 Days
Evidence Blueprint
A machine-learning approach for differentiating borderline personality disorder from community participants with brain-wide functional connectivity
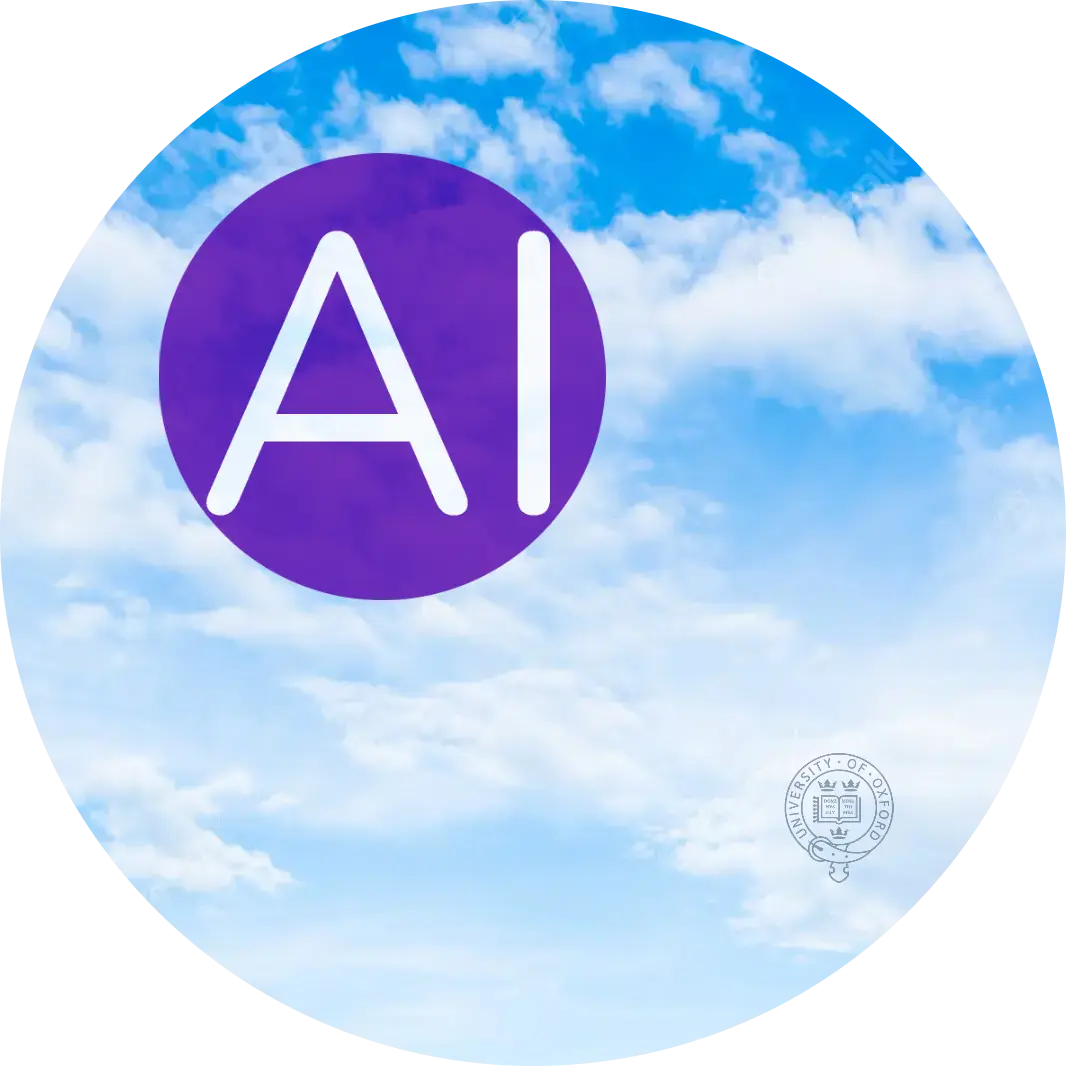
☊ AI-Driven Related Evidence Nodes
(recent articles with at least 5 words in title)
More Evidence
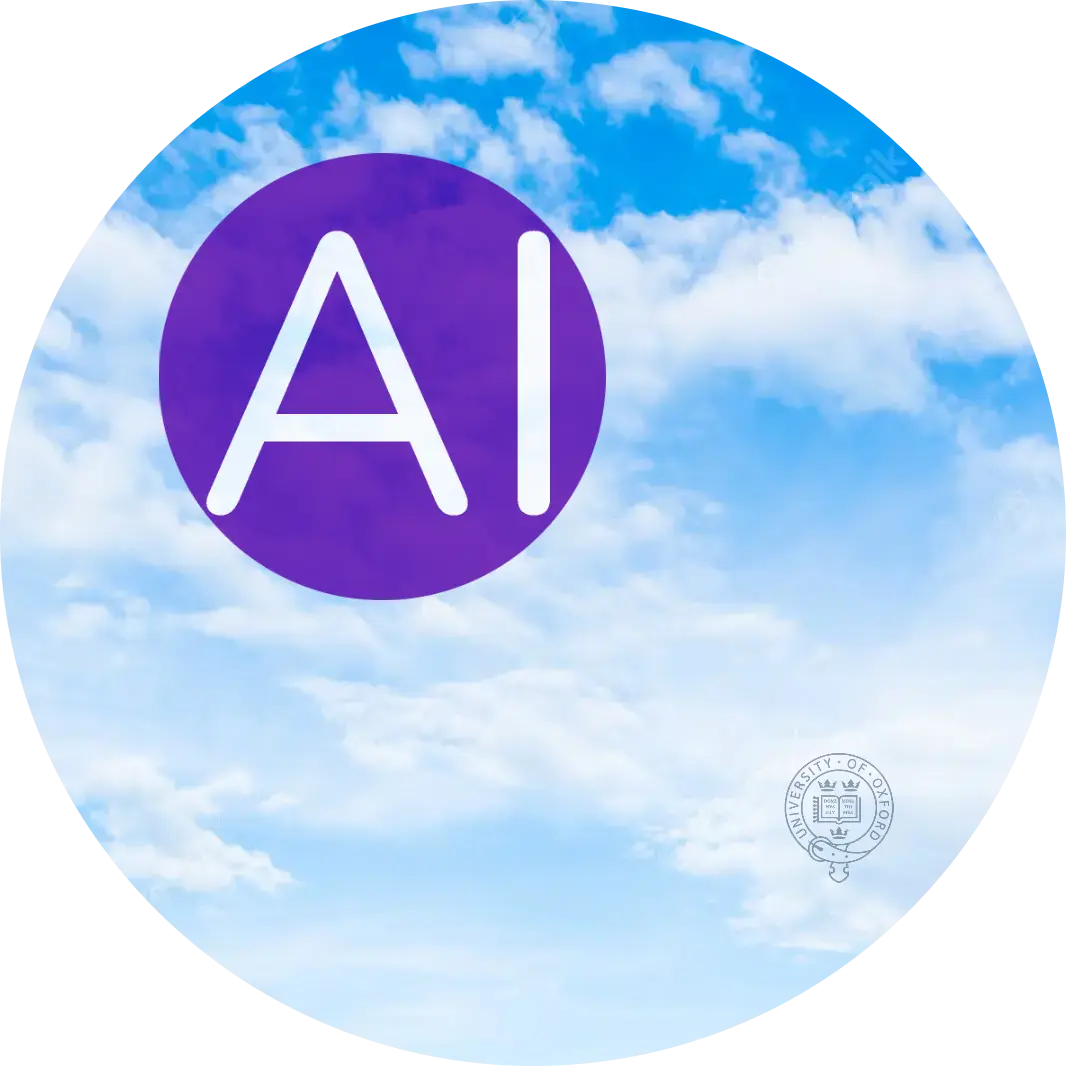