Evidence
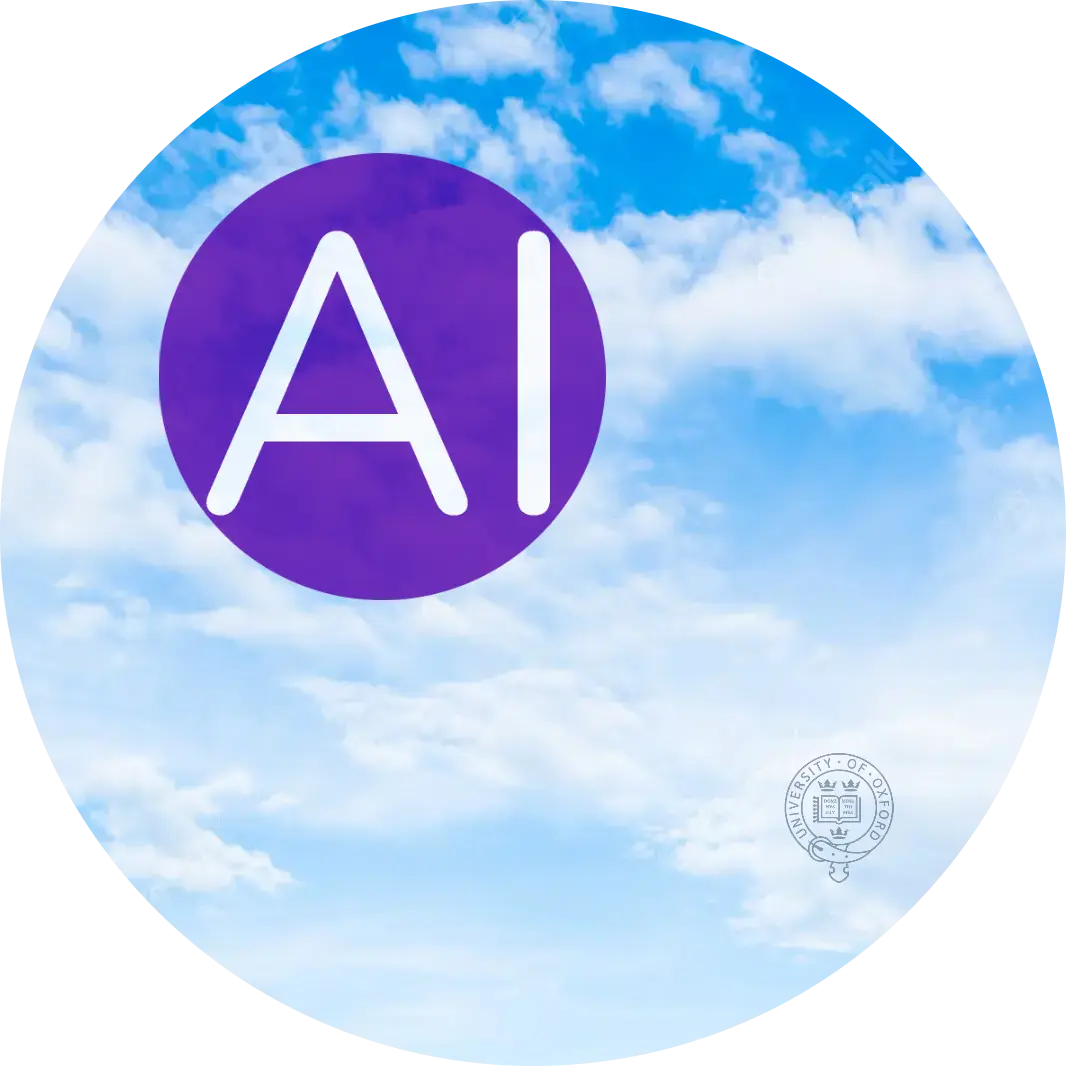
Front Aging Neurosci. 2024 Aug 12;16:1410544. doi: 10.3389/fnagi.2024.1410544. eCollection 2024.
ABSTRACT
INTRODUCTION: Alzheimer’s disease (AD) is highly heterogeneous, with substantial individual variabilities in clinical progression and neurobiology. Amyloid deposition has been thought to drive cognitive decline and thus a major contributor to the variations in cognitive deterioration in AD. However, the clinical heterogeneity of patients with early symptomatic AD (mild cognitive impairment or mild dementia due to AD) already with evidence of amyloid abnormality in the brain is still unknown.
METHODS: Participants with a baseline diagnosis of mild cognitive impairment or mild dementia, a positive amyloid-PET scan, and more than one follow-up Alzheimer’s Disease Assessment Scale-Cognitive Subscale-13 (ADAS-Cog-13) administration within a period of 5-year follow-up were selected from the Alzheimer’s Disease Neuroimaging Initiative database (n = 421; age = 73±7; years of education = 16 ± 3; percentage of female gender = 43%; distribution of APOE4 carriers = 68%). A non-parametric k-means longitudinal clustering analysis in the context of the ADAS-Cog-13 data was performed to identify cognitive subtypes.
RESULTS: We found a highly variable profile of cognitive decline among patients with early AD and identified 4 clusters characterized by distinct rates of cognitive progression. Among the groups there were significant differences in the magnitude of rates of changes in other cognitive and functional outcomes, clinical progression from mild cognitive impairment to dementia, and changes in markers presumed to reflect neurodegeneration and neuronal injury. A nomogram based on a simplified logistic regression model predicted steep cognitive trajectory with an AUC of 0.912 (95% CI: 0.88 – 0.94). Simulation of clinical trials suggested that the incorporation of the nomogram into enrichment strategies would reduce the required sample sizes from 926.8 (95% CI: 822.6 – 1057.5) to 400.9 (95% CI: 306.9 – 516.8).
DISCUSSION: Our findings show usefulness in the stratification of patients in early AD and may thus increase the chances of finding a treatment for future AD clinical trials.
PMID:39193492 | PMC:PMC11348433 | DOI:10.3389/fnagi.2024.1410544



Estimated reading time: 5 minute(s)
Latest: Psychiatryai.com #RAISR4D Evidence

Cool Evidence: Engaging Young People and Students in Real-World Evidence

Real-Time Evidence Search [Psychiatry]

AI Research

Characterizing the clinical heterogeneity of early symptomatic Alzheimer’s disease: a data-driven machine learning approach
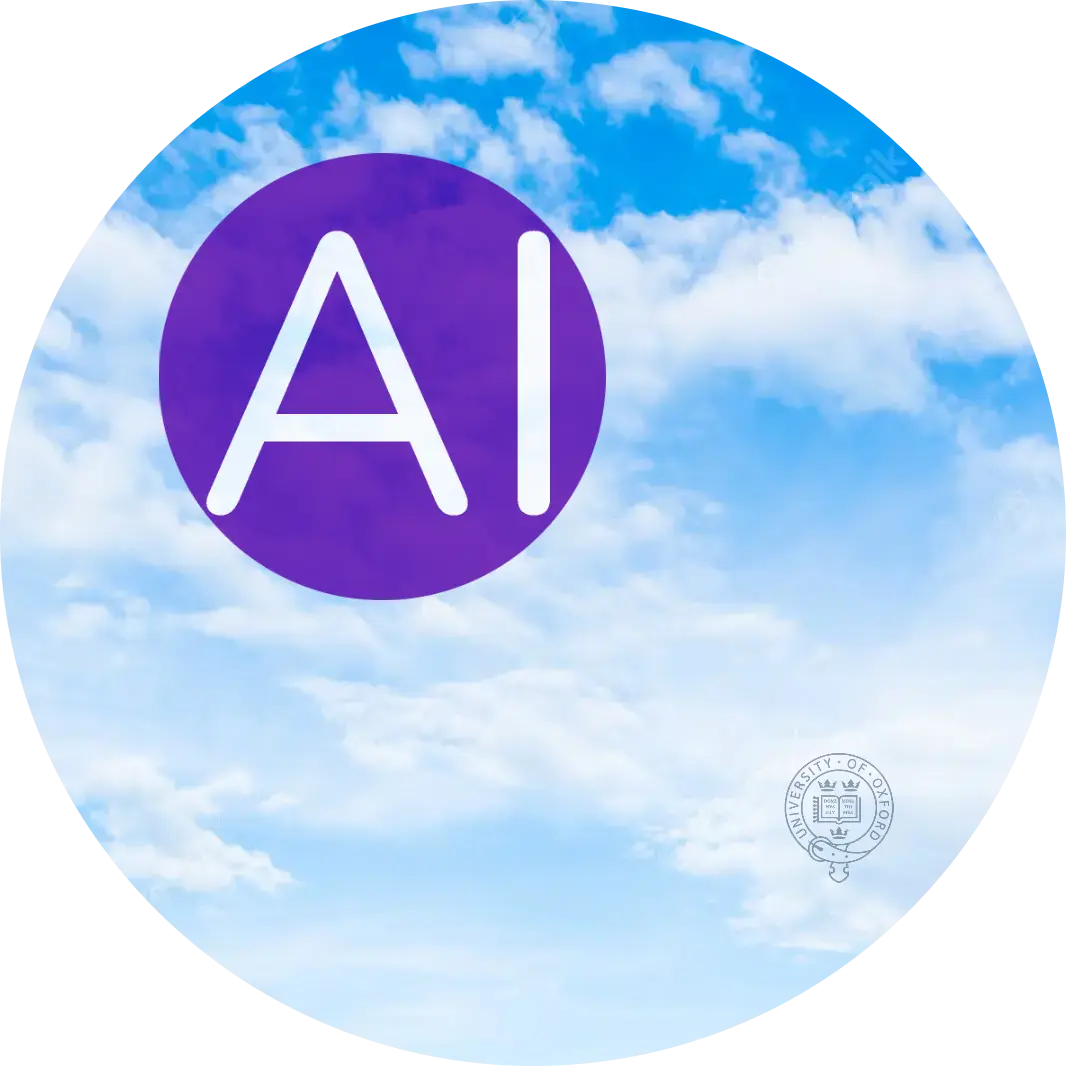
🌐 90 Days
Evidence Blueprint
Characterizing the clinical heterogeneity of early symptomatic Alzheimer’s disease: a data-driven machine learning approach
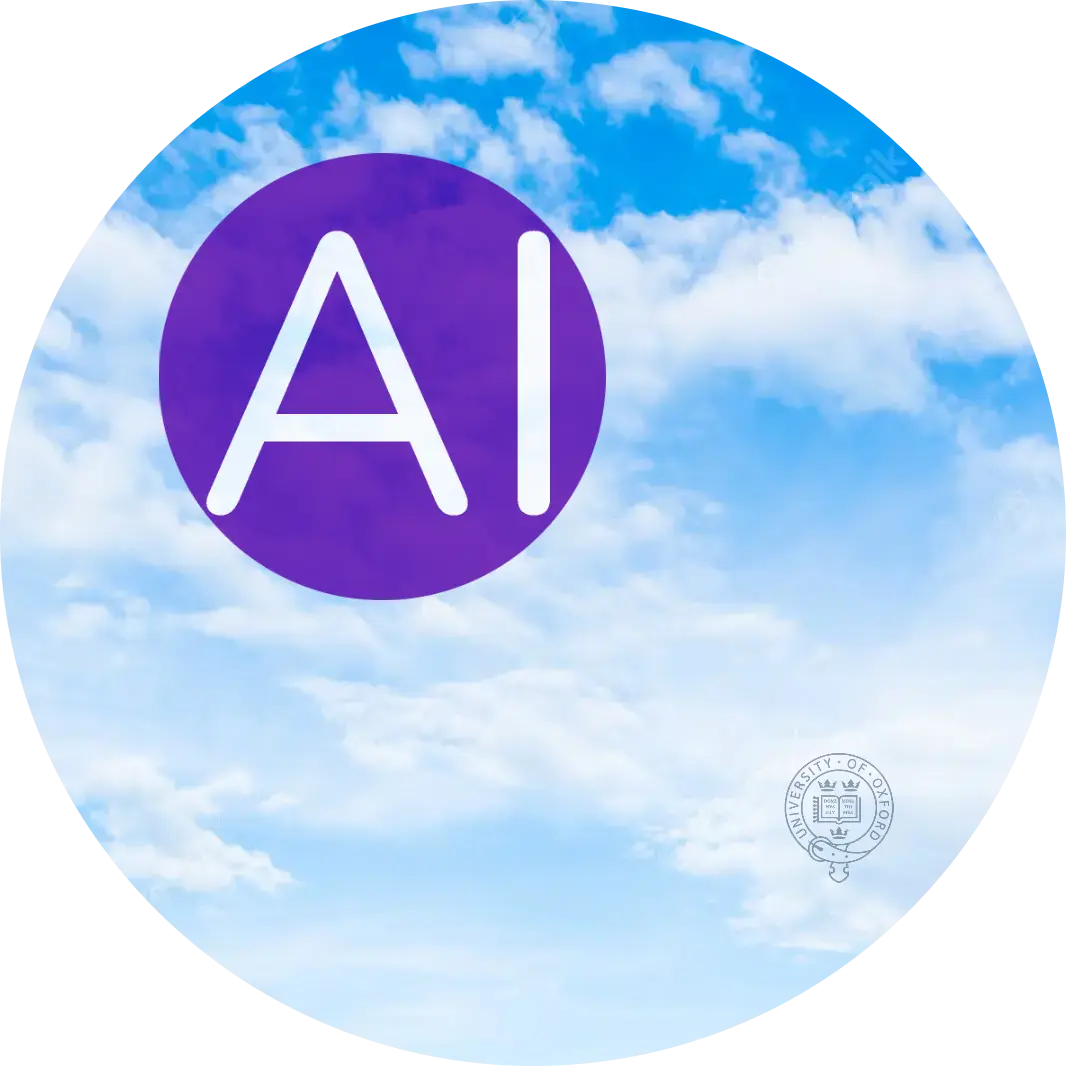
☊ AI-Driven Related Evidence Nodes
(recent articles with at least 5 words in title)
More Evidence
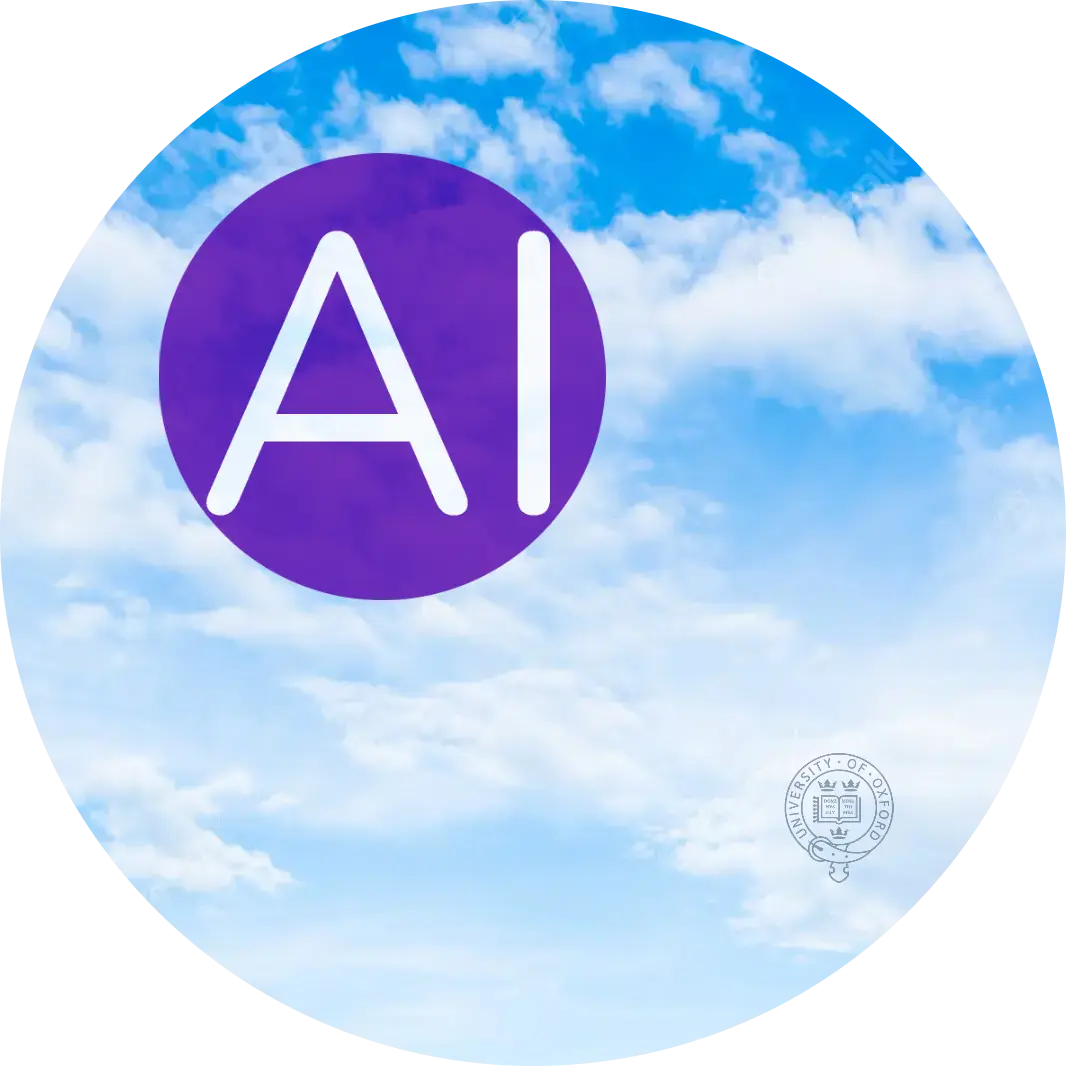