Evidence
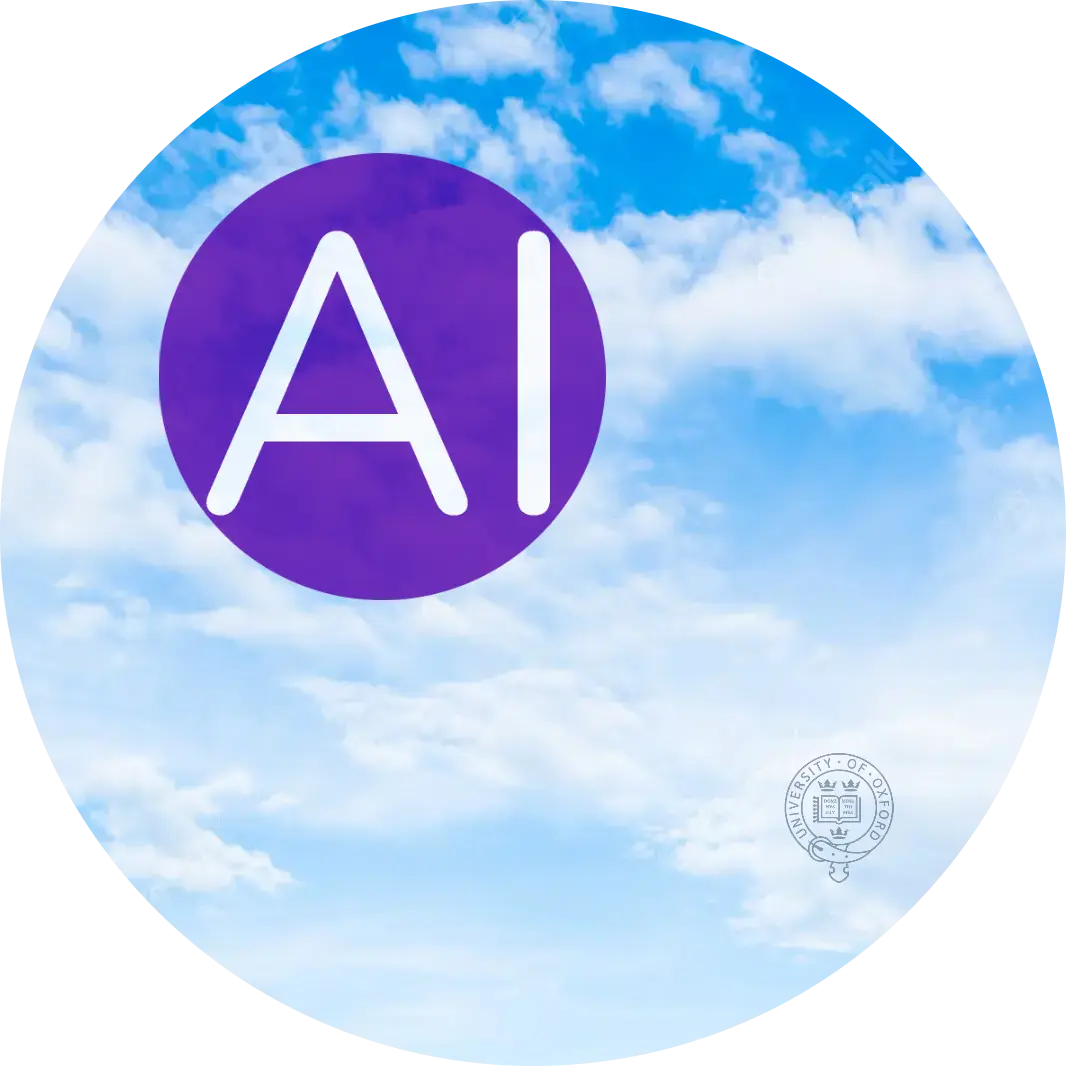
Eur Radiol Exp. 2024 Jul 15;8(1):80. doi: 10.1186/s41747-024-00478-6.
ABSTRACT
INTRODUCTION: Breast arterial calcifications (BAC) are common incidental findings on routine mammograms, which have been suggested as a sex-specific biomarker of cardiovascular disease (CVD) risk. Previous work showed the efficacy of a pretrained convolutional network (CNN), VCG16, for automatic BAC detection. In this study, we further tested the method by a comparative analysis with other ten CNNs.
MATERIAL AND METHODS: Four-view standard mammography exams from 1,493 women were included in this retrospective study and labeled as BAC or non-BAC by experts. The comparative study was conducted using eleven pretrained convolutional networks (CNNs) with varying depths from five architectures including Xception, VGG, ResNetV2, MobileNet, and DenseNet, fine-tuned for the binary BAC classification task. Performance evaluation involved area under the receiver operating characteristics curve (AUC-ROC) analysis, F1-score (harmonic mean of precision and recall), and generalized gradient-weighted class activation mapping (Grad-CAM++) for visual explanations.
RESULTS: The dataset exhibited a BAC prevalence of 194/1,493 women (13.0%) and 581/5,972 images (9.7%). Among the retrained models, VGG, MobileNet, and DenseNet demonstrated the most promising results, achieving AUC-ROCs > 0.70 in both training and independent testing subsets. In terms of testing F1-score, VGG16 ranked first, higher than MobileNet (0.51) and VGG19 (0.46). Qualitative analysis showed that the Grad-CAM++ heatmaps generated by VGG16 consistently outperformed those produced by others, offering a finer-grained and discriminative localization of calcified regions within images.
CONCLUSION: Deep transfer learning showed promise in automated BAC detection on mammograms, where relatively shallow networks demonstrated superior performances requiring shorter training times and reduced resources.
RELEVANCE STATEMENT: Deep transfer learning is a promising approach to enhance reporting BAC on mammograms and facilitate developing efficient tools for cardiovascular risk stratification in women, leveraging large-scale mammographic screening programs.
KEY POINTS: • We tested different pretrained convolutional networks (CNNs) for BAC detection on mammograms. • VGG and MobileNet demonstrated promising performances, outperforming their deeper, more complex counterparts. • Visual explanations using Grad-CAM++ highlighted VGG16’s superior performance in localizing BAC.
PMID:39004645 | DOI:10.1186/s41747-024-00478-6



Estimated reading time: 6 minute(s)
Latest: Psychiatryai.com #RAISR4D Evidence

Cool Evidence: Engaging Young People and Students in Real-World Evidence

Real-Time Evidence Search [Psychiatry]

AI Research

Deep transfer learning for detection of breast arterial calcifications on mammograms: a comparative study
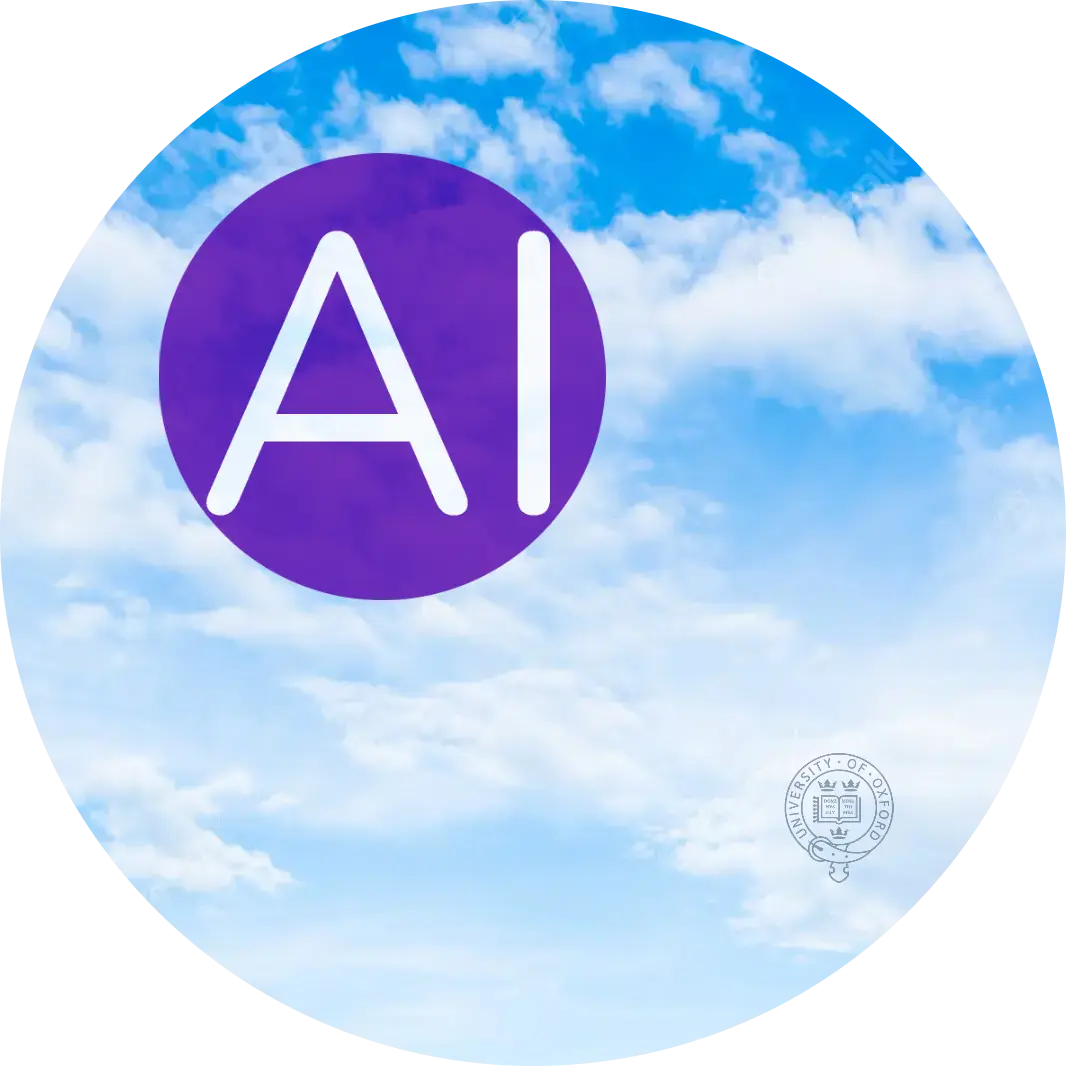
🌐 90 Days
Evidence Blueprint
Deep transfer learning for detection of breast arterial calcifications on mammograms: a comparative study
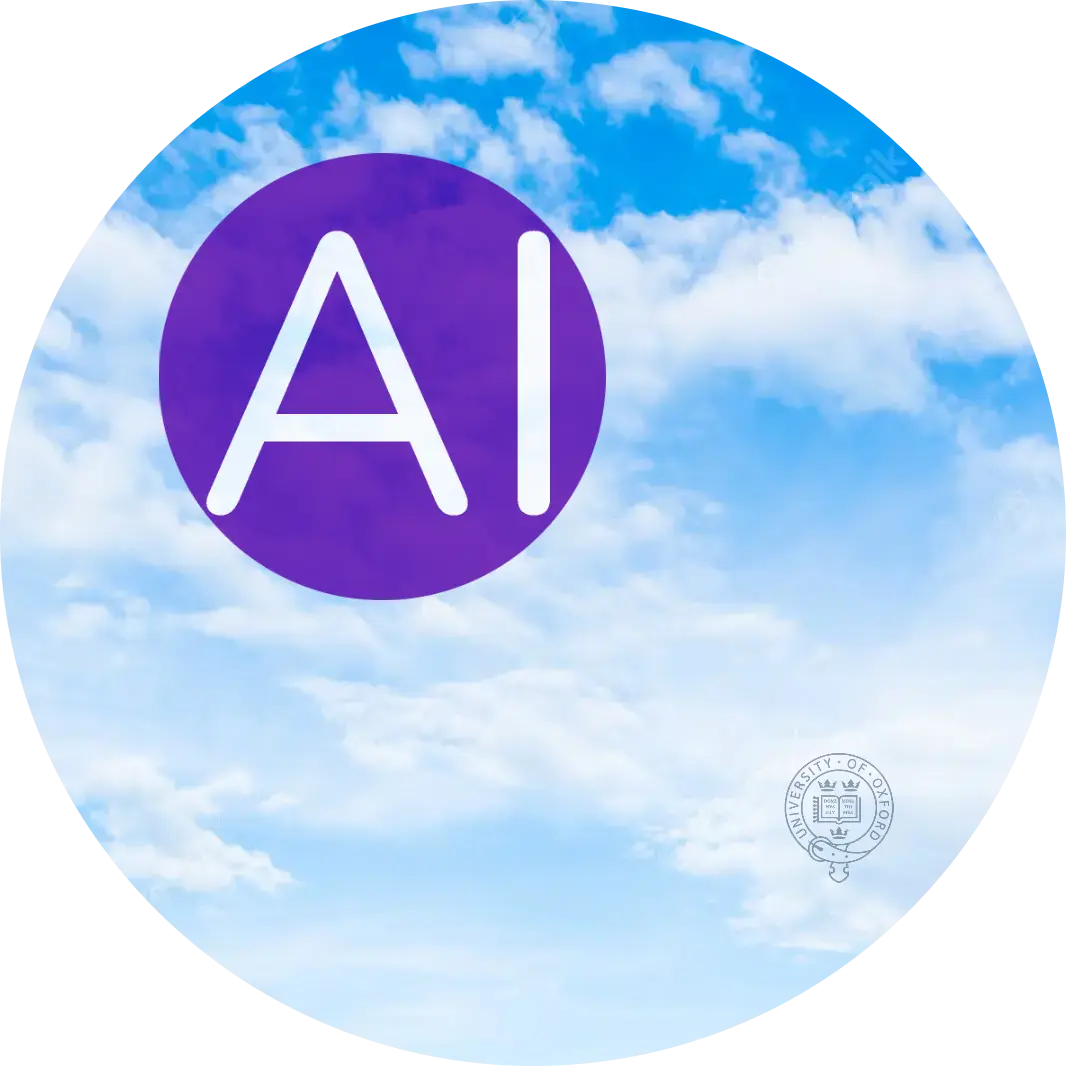
☊ AI-Driven Related Evidence Nodes
(recent articles with at least 5 words in title)
More Evidence
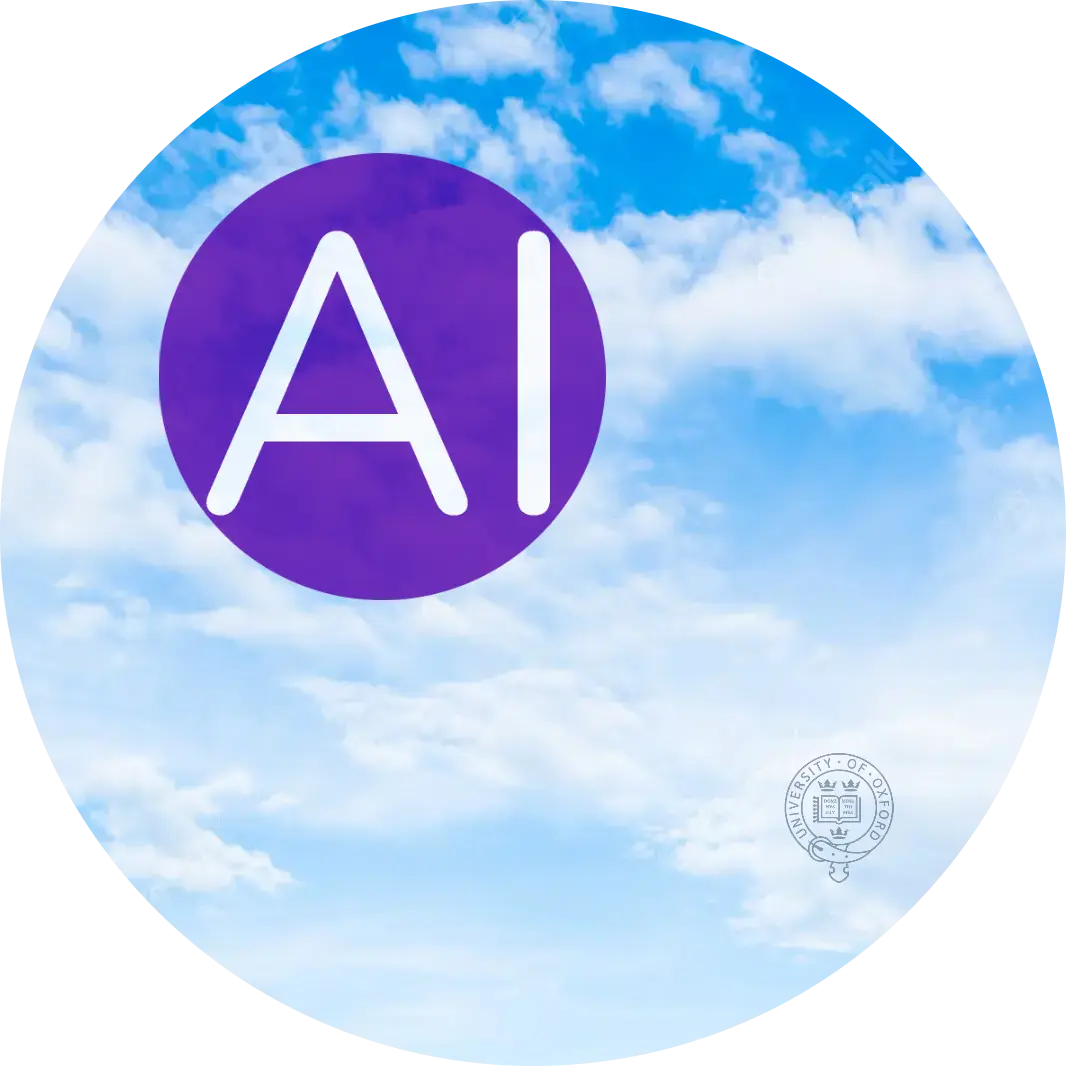