Evidence
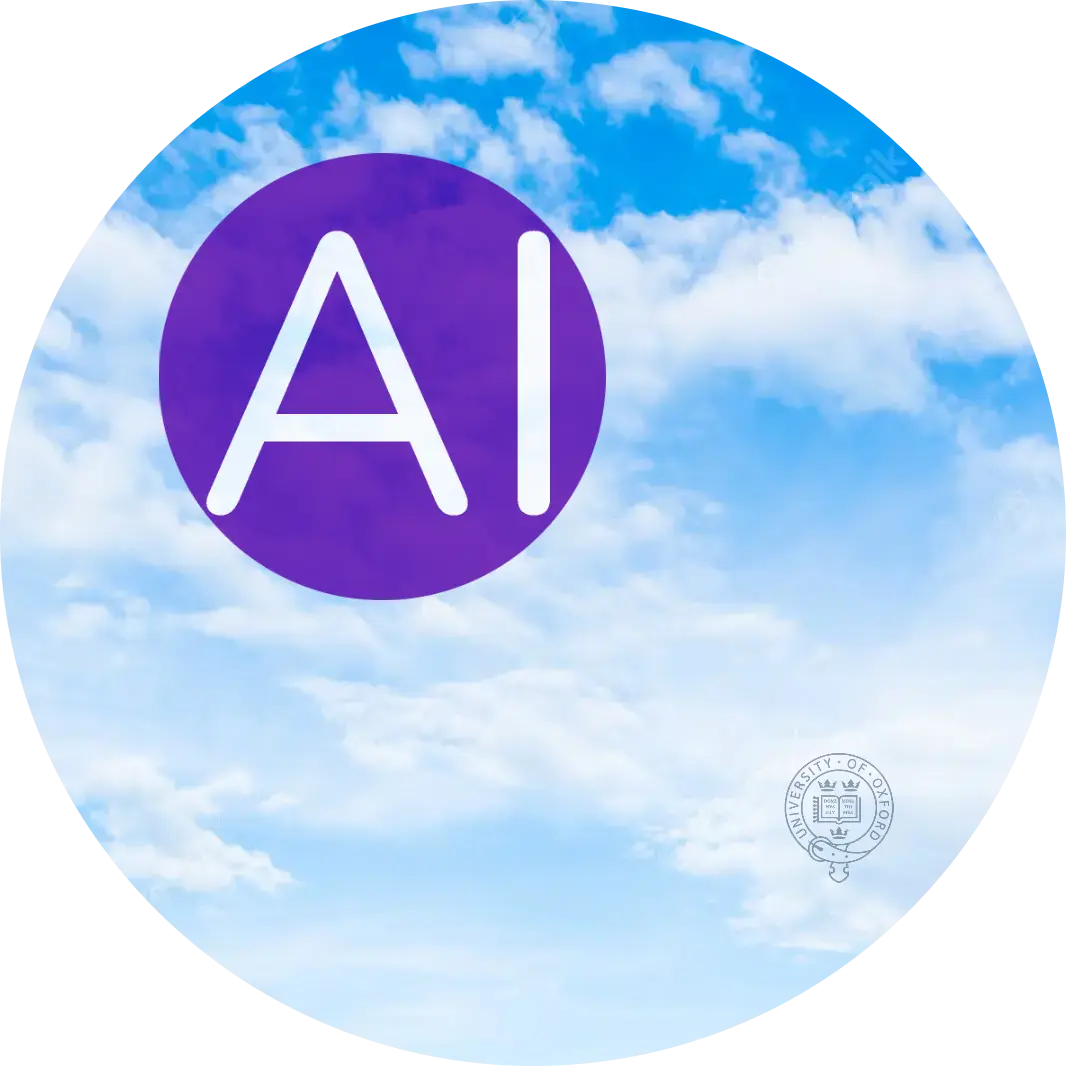
Psychol Med. 2023 May 22:1-11. doi: 10.1017/S0033291723001319. Online ahead of print.
ABSTRACT
BACKGROUND: Individuals with bipolar disorder are commonly correctly diagnosed a decade after symptom onset. Machine learning techniques may aid in early recognition and reduce the disease burden. As both individuals at risk and those with a manifest disease display structural brain markers, structural magnetic resonance imaging may provide relevant classification features.
METHODS: Following a pre-registered protocol, we trained linear support vector machine (SVM) to classify individuals according to their estimated risk for bipolar disorder using regional cortical thickness of help-seeking individuals from seven study sites (N = 276). We estimated the risk using three state-of-the-art assessment instruments (BPSS-P, BARS, EPIbipolar).
RESULTS: For BPSS-P, SVM achieved a fair performance of Cohen’s κ of 0.235 (95% CI 0.11-0.361) and a balanced accuracy of 63.1% (95% CI 55.9-70.3) in the 10-fold cross-validation. In the leave-one-site-out cross-validation, the model performed with a Cohen’s κ of 0.128 (95% CI -0.069 to 0.325) and a balanced accuracy of 56.2% (95% CI 44.6-67.8). BARS and EPIbipolar could not be predicted. In post hoc analyses, regional surface area, subcortical volumes as well as hyperparameter optimization did not improve the performance.
CONCLUSIONS: Individuals at risk for bipolar disorder, as assessed by BPSS-P, display brain structural alterations that can be detected using machine learning. The achieved performance is comparable to previous studies which attempted to classify patients with manifest disease and healthy controls. Unlike previous studies of bipolar risk, our multicenter design permitted a leave-one-site-out cross-validation. Whole-brain cortical thickness seems to be superior to other structural brain features.
PMID:37212052 | DOI:10.1017/S0033291723001319



Estimated reading time: 5 minute(s)
Latest: Psychiatryai.com #RAISR4D

Cool Evidence: Engaging Young People and Students in Real-World Evidence ☀️

Real-Time Evidence Search [Psychiatry]

AI Research [Andisearch.com]

Prediction of estimated risk for bipolar disorder using machine learning and structural MRI features
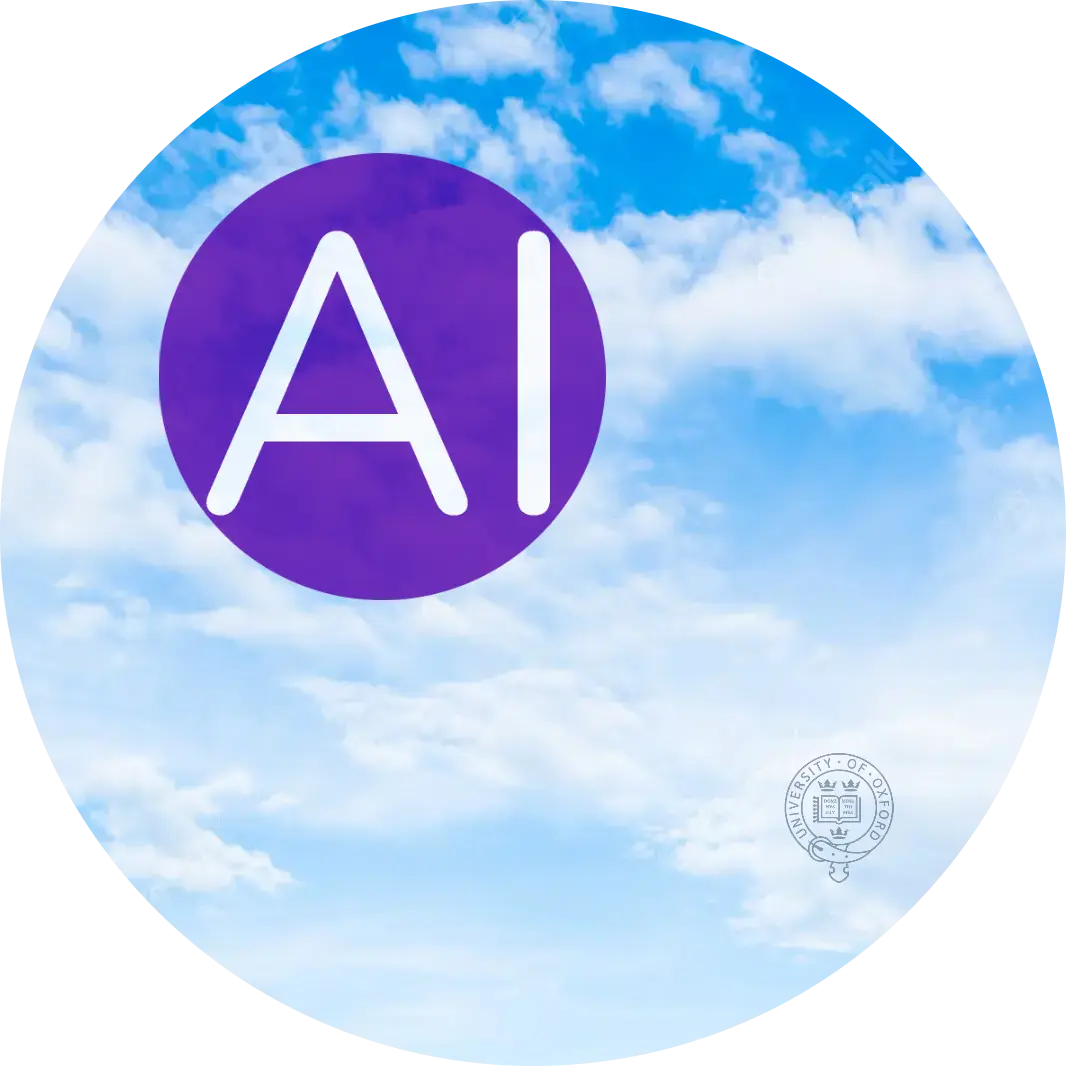
🌐 90 Days
Evidence Blueprint
Prediction of estimated risk for bipolar disorder using machine learning and structural MRI features
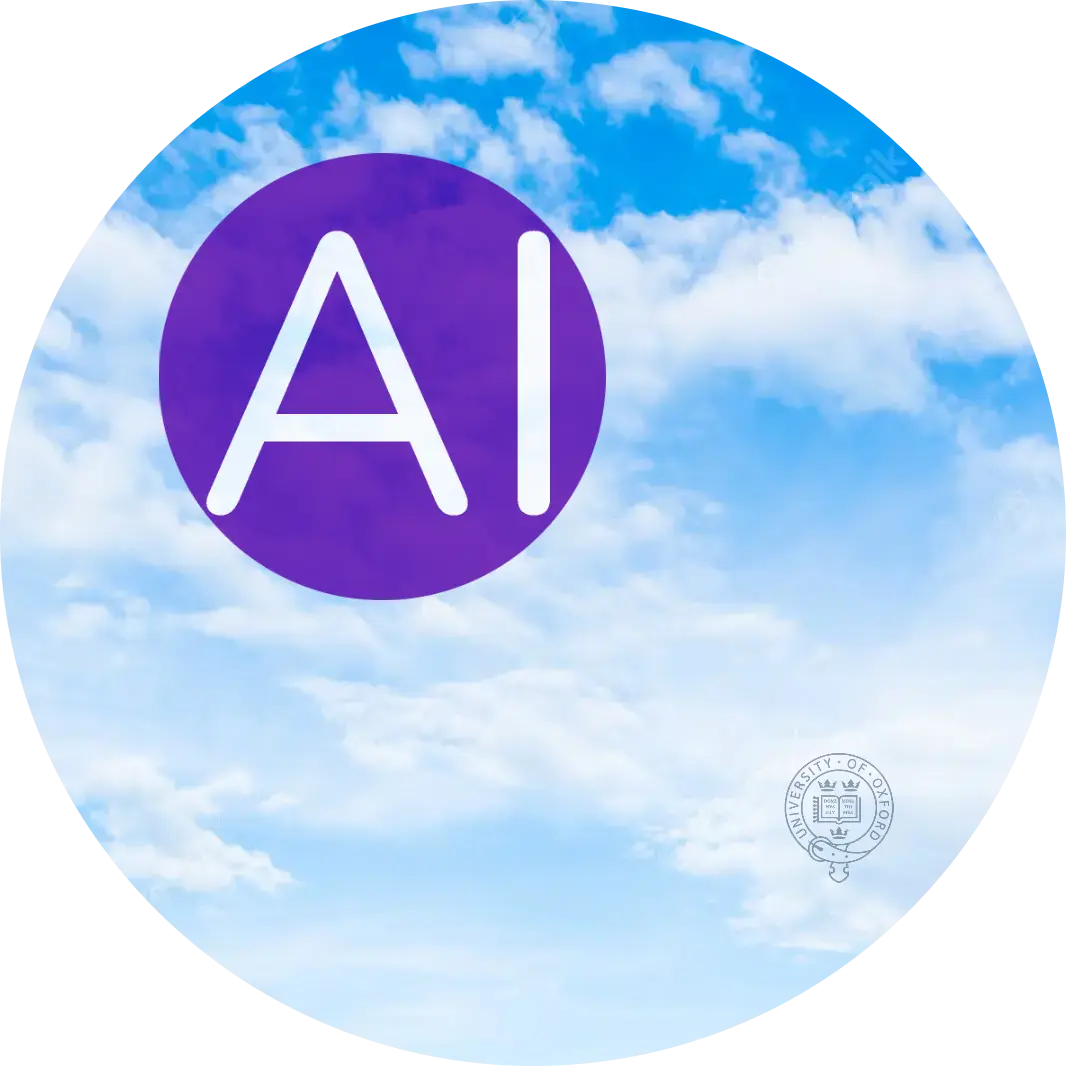
☊ AI-Driven Related Evidence Nodes
(recent articles with at least 5 words in title)
More Evidence
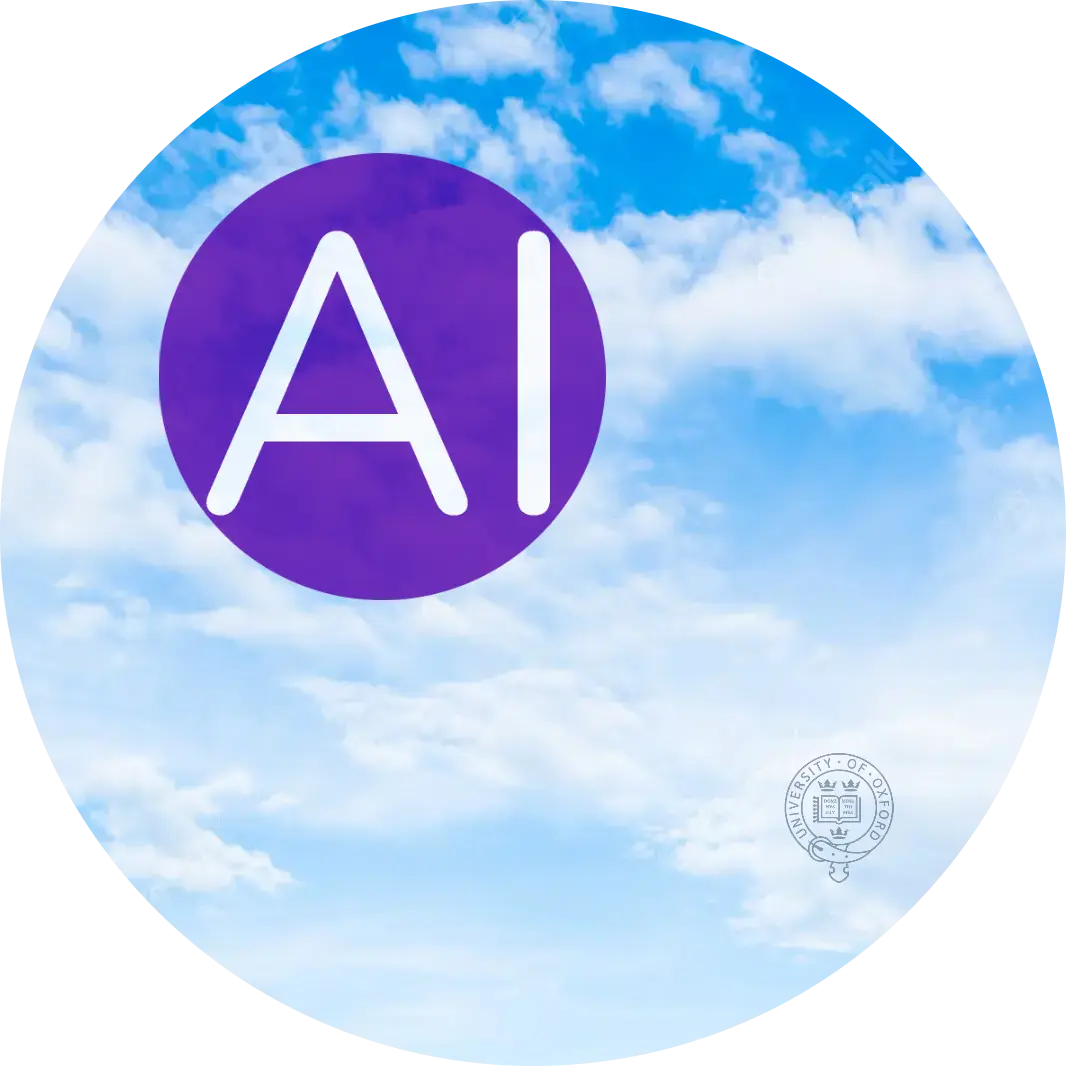