Evidence
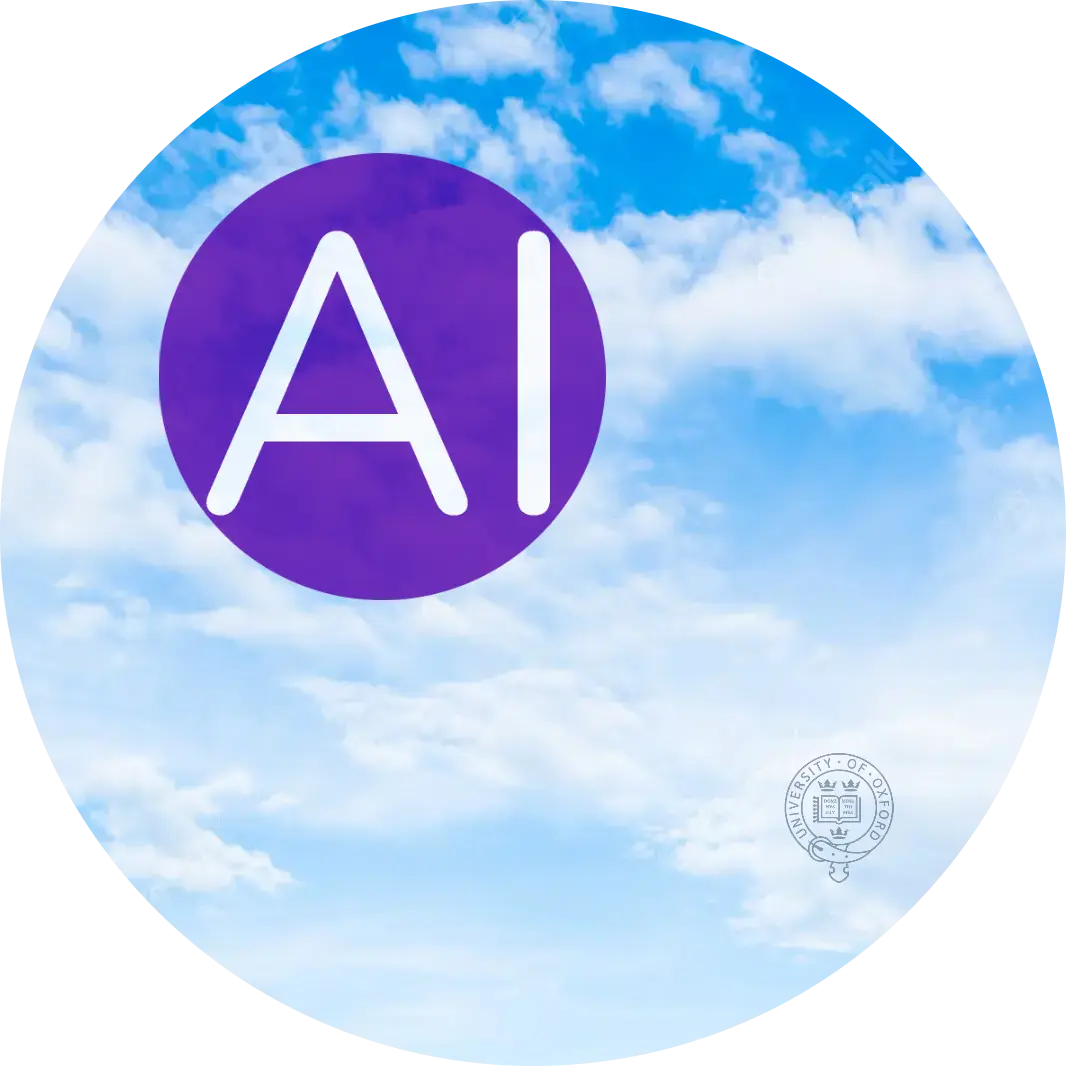
Comput Methods Programs Biomed. 2024 Aug 16;257:108378. doi: 10.1016/j.cmpb.2024.108378. Online ahead of print.
ABSTRACT
BACKGROUND AND OBJECTIVE: Alzheimer’s disease (AD) is one of the leading causes of dementia, affecting the world’s population at a growing rate. The preclinical stage of AD lasts over a decade, hence understanding AD-related early neuropathological effects on brain function at this stage facilitates early detection of the disease.
METHODS: Resting-state functional magnetic resonance imaging (rs-fMRI) has been a powerful tool for understanding brain function, and it has been widely used in AD research. In this study, we apply Recurrence Quantification Analysis (RQA) on rs-fMRI images of 4-months (4 m) and 6-months-old (6 m) TgF344-AD rats and WT littermates to identify changes related to the AD phenotype and aging. RQA has been focused on areas of the default mode-like network (DMLN) and was performed based on Recurrence Plots (RP). RP is a mathematical representation of any dynamical system that evolves over time as a set of its state recurrences. In this paper, RPs were extracted in order to identify the affected regions of the DMLN at very early stages of AD.
RESULTS: Using the RQA approach, we identified significant changes related to the AD phenotype at 4 m and/or 6 m in several areas of the rat DMLN including the BFB, Hippocampal fields CA1 and CA3, CG1, CG2, PrL, PtA, RSC, TeA, V1, V2. In addition, with age, brain activity of WT rats showed less predictability, while the AD rats presented reduced decline of predictability.
CONCLUSIONS: The results of this study demonstrate that RQA of rs-fMRI data is a potent approach that can detect subtle changes which might be missed by other methodologies due to the brain’s non-linear dynamics. Moreover, this study provides helpful information about specific areas involved in AD pathology at very early stages of the disease in a very promising rat model of AD. Our results provide valuable information for the development of early detection methods and novel diagnosis tools for AD.
PMID:39260164 | DOI:10.1016/j.cmpb.2024.108378



Estimated reading time: 6 minute(s)
Latest: Psychiatryai.com #RAISR4D Evidence

Cool Evidence: Engaging Young People and Students in Real-World Evidence

Real-Time Evidence Search [Psychiatry]

AI Research

Recurrence quantification analysis of rs-fMRI data: A method to detect subtle changes in the TgF344-AD rat model
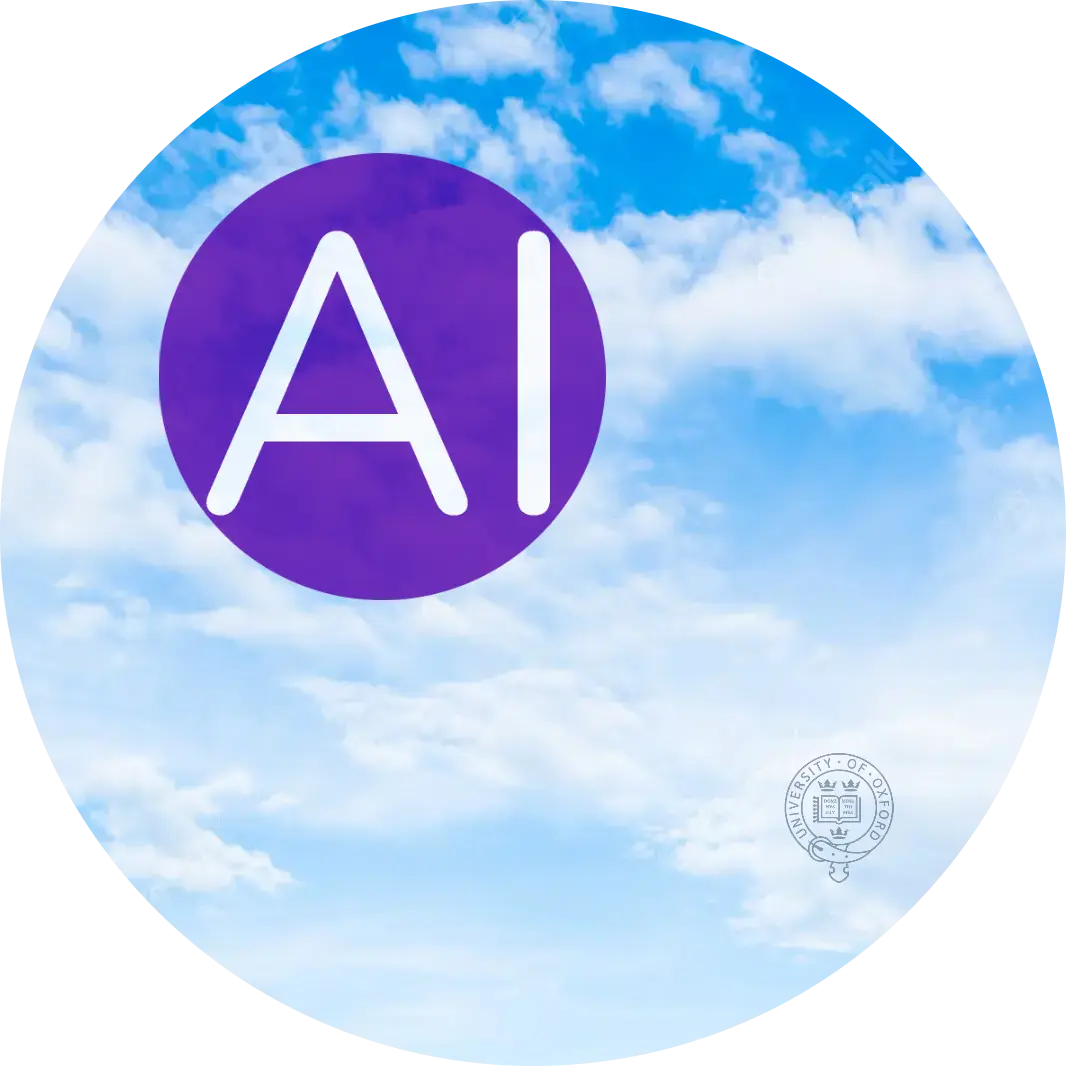
🌐 90 Days
Evidence Blueprint
Recurrence quantification analysis of rs-fMRI data: A method to detect subtle changes in the TgF344-AD rat model
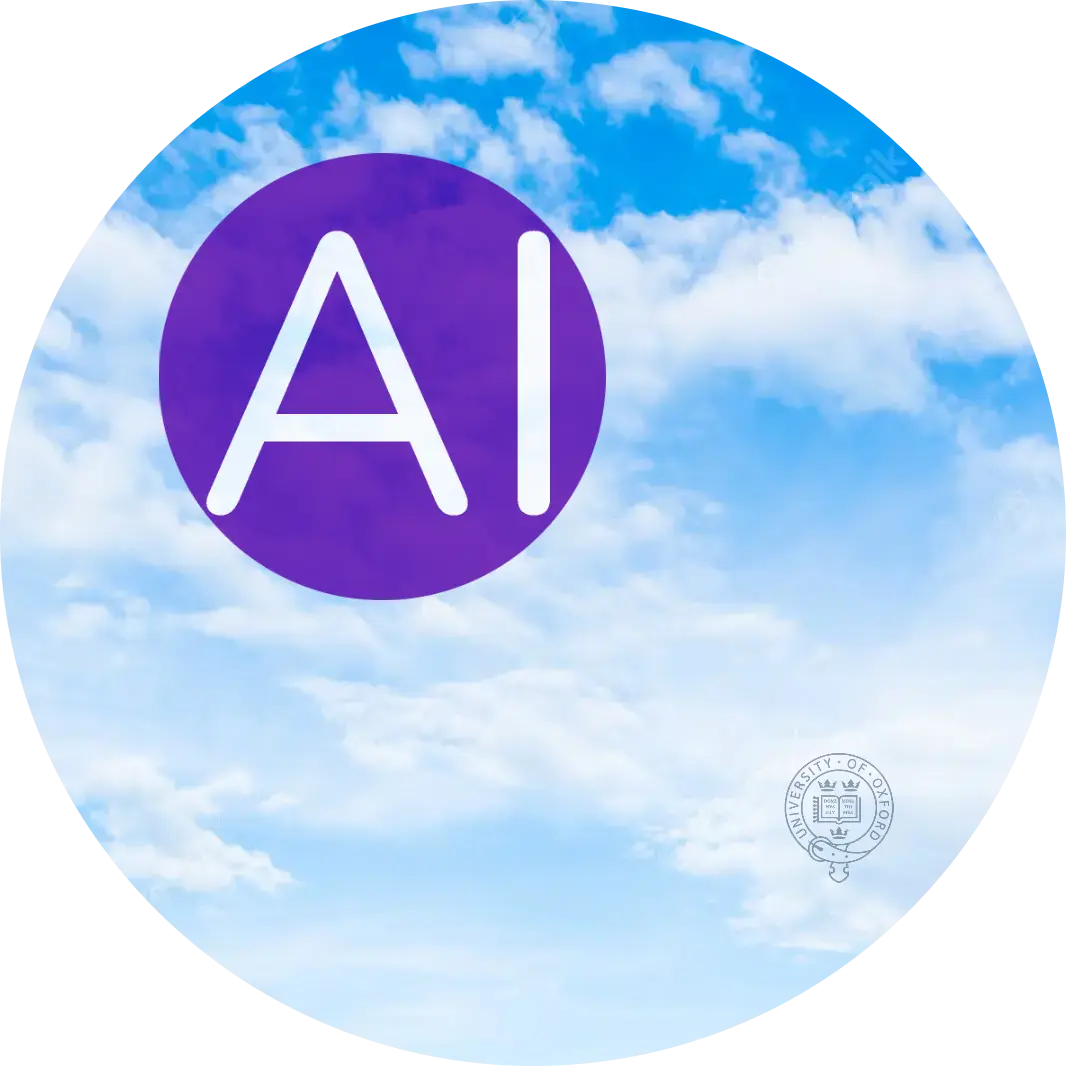
☊ AI-Driven Related Evidence Nodes
(recent articles with at least 5 words in title)
More Evidence
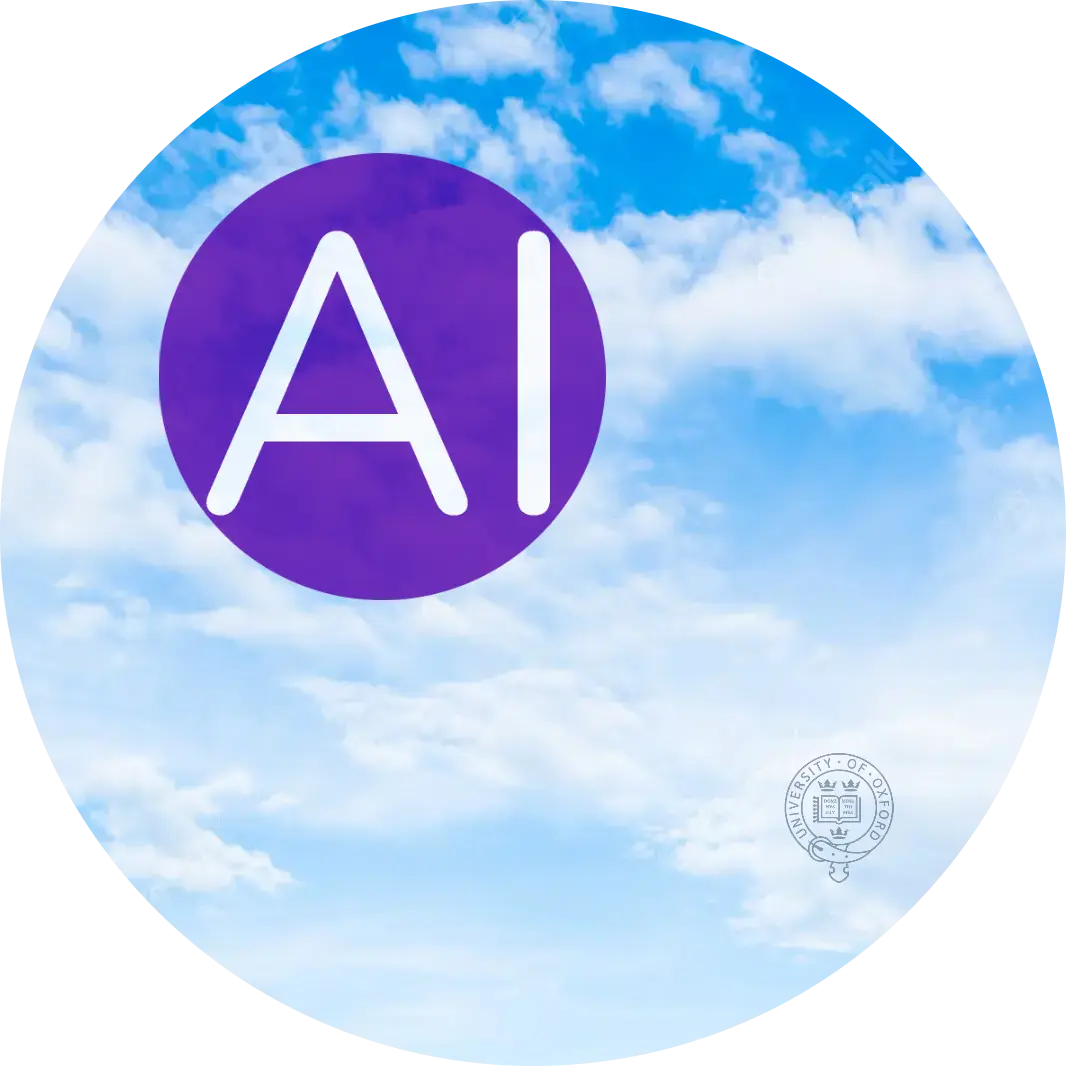