Evidence
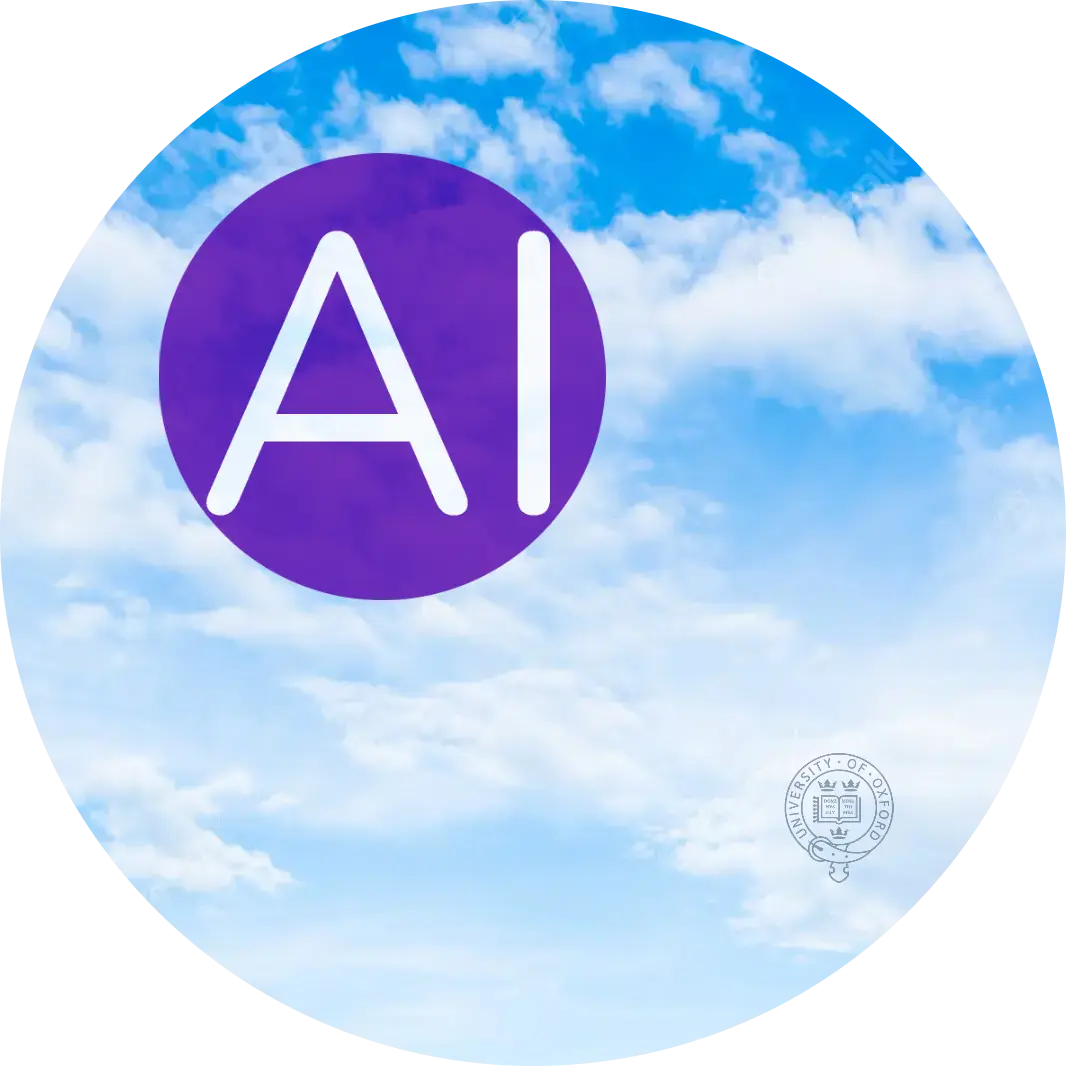
Eur Heart J Digit Health. 2024 Feb 19;5(3):247-259. doi: 10.1093/ehjdh/ztae014. eCollection 2024 May.
ABSTRACT
AIMS: Electrocardiogram (ECG) is widely considered the primary test for evaluating cardiovascular diseases. However, the use of artificial intelligence (AI) to advance these medical practices and learn new clinical insights from ECGs remains largely unexplored. We hypothesize that AI models with a specific design can provide fine-grained interpretation of ECGs to advance cardiovascular diagnosis, stratify mortality risks, and identify new clinically useful information.
METHODS AND RESULTS: Utilizing a data set of 2 322 513 ECGs collected from 1 558 772 patients with 7 years follow-up, we developed a deep-learning model with state-of-the-art granularity for the interpretable diagnosis of cardiac abnormalities, gender identification, and hypertension screening solely from ECGs, which are then used to stratify the risk of mortality. The model achieved the area under the receiver operating characteristic curve (AUC) scores of 0.998 (95% confidence interval (CI), 0.995-0.999), 0.964 (95% CI, 0.963-0.965), and 0.839 (95% CI, 0.837-0.841) for the three diagnostic tasks separately. Using ECG-predicted results, we find high risks of mortality for subjects with sinus tachycardia (adjusted hazard ratio (HR) of 2.24, 1.96-2.57), and atrial fibrillation (adjusted HR of 2.22, 1.99-2.48). We further use salient morphologies produced by the deep-learning model to identify key ECG leads that achieved similar performance for the three diagnoses, and we find that the V1 ECG lead is important for hypertension screening and mortality risk stratification of hypertensive cohorts, with an AUC of 0.816 (0.814-0.818) and a univariate HR of 1.70 (1.61-1.79) for the two tasks separately.
CONCLUSION: Using ECGs alone, our developed model showed cardiologist-level accuracy in interpretable cardiac diagnosis and the advancement in mortality risk stratification. In addition, it demonstrated the potential to facilitate clinical knowledge discovery for gender and hypertension detection which are not readily available.
PMID:38774384 | PMC:PMC11104458 | DOI:10.1093/ehjdh/ztae014



Estimated reading time: 5 minute(s)
Latest: Psychiatryai.com #RAISR4D Evidence

Cool Evidence: Engaging Young People and Students in Real-World Evidence

Real-Time Evidence Search [Psychiatry]

AI Research

Decoding 2.3 million ECGs: interpretable deep learning for advancing cardiovascular diagnosis and mortality risk stratification
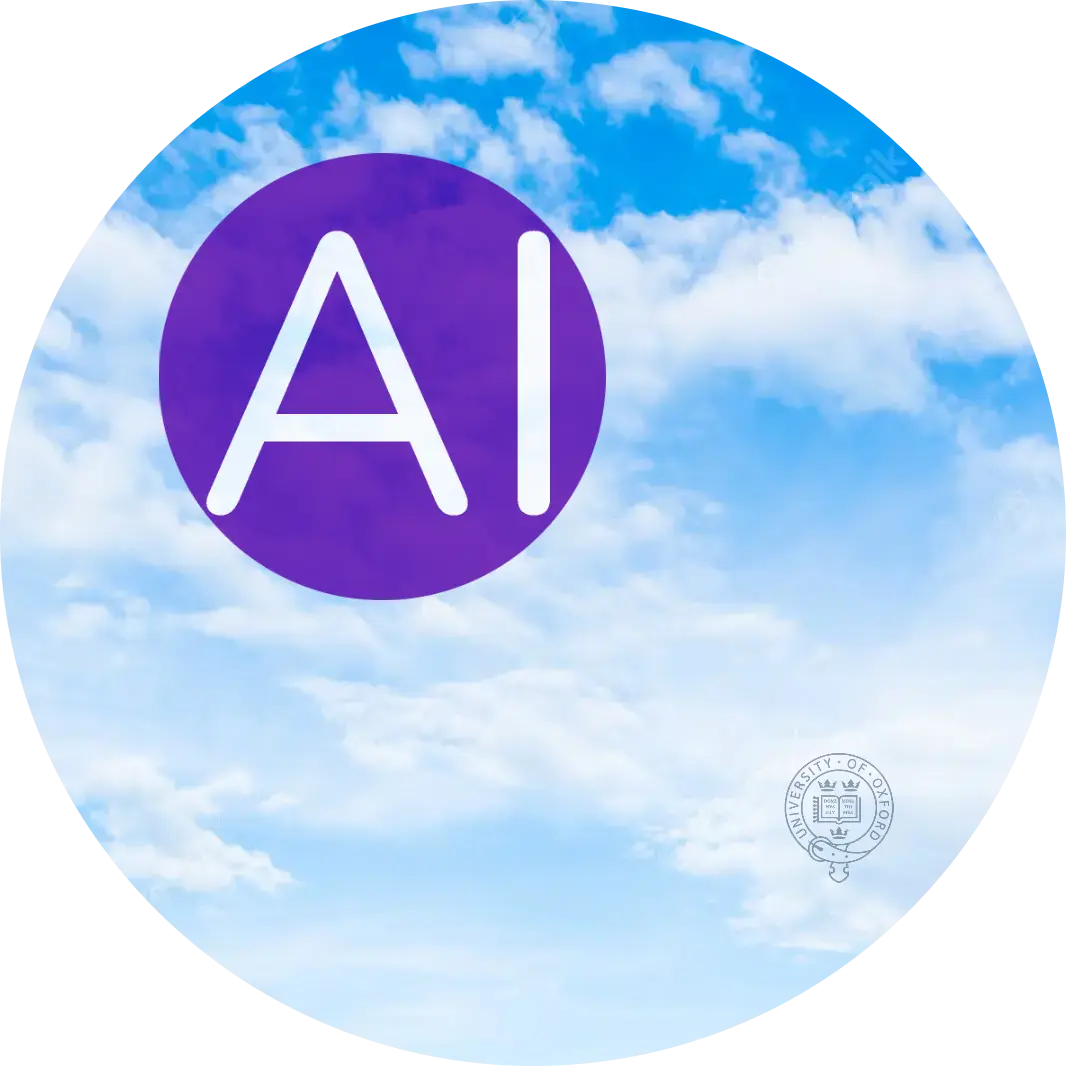
🌐 90 Days
Evidence Blueprint
Decoding 2.3 million ECGs: interpretable deep learning for advancing cardiovascular diagnosis and mortality risk stratification
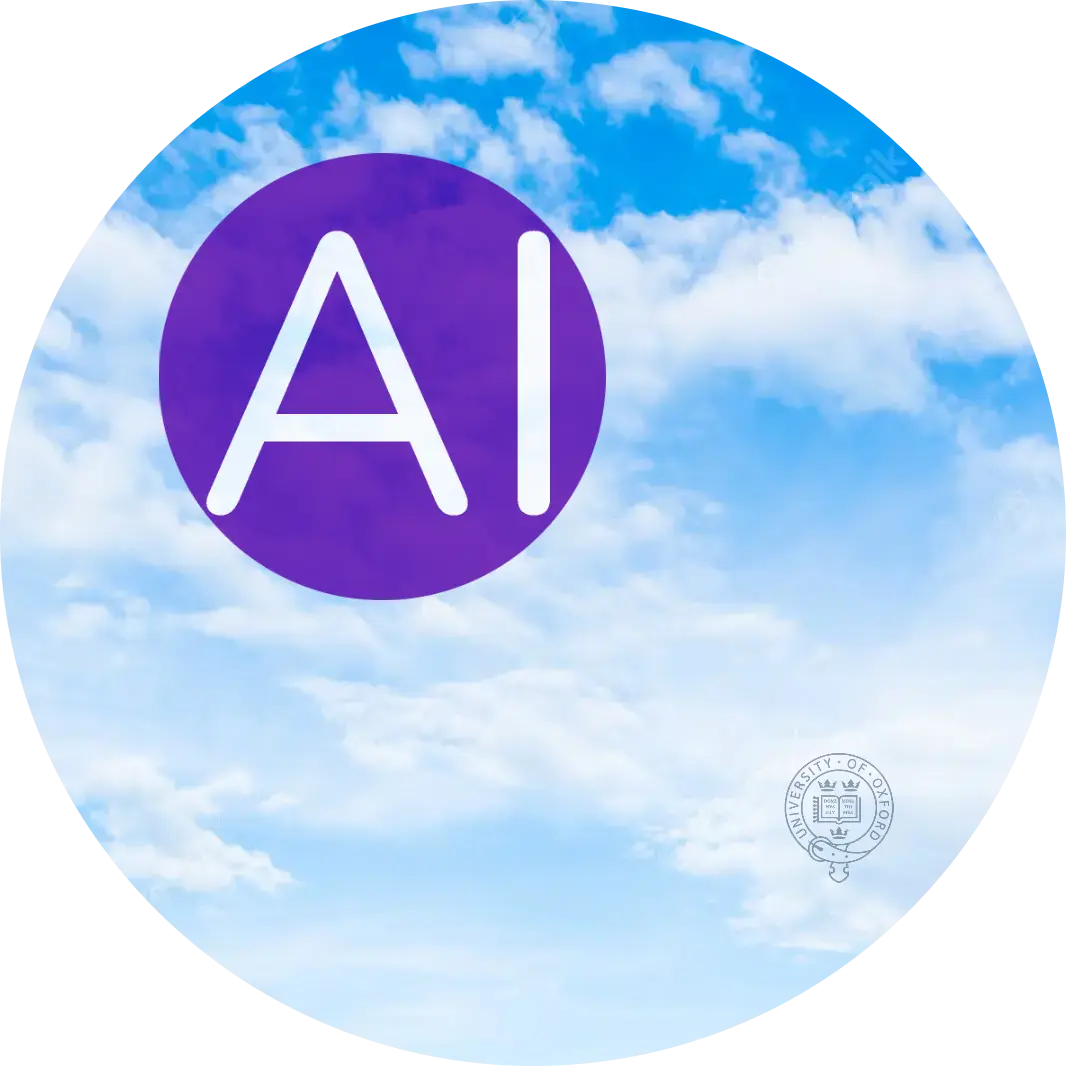
☊ AI-Driven Related Evidence Nodes
(recent articles with at least 5 words in title)
More Evidence
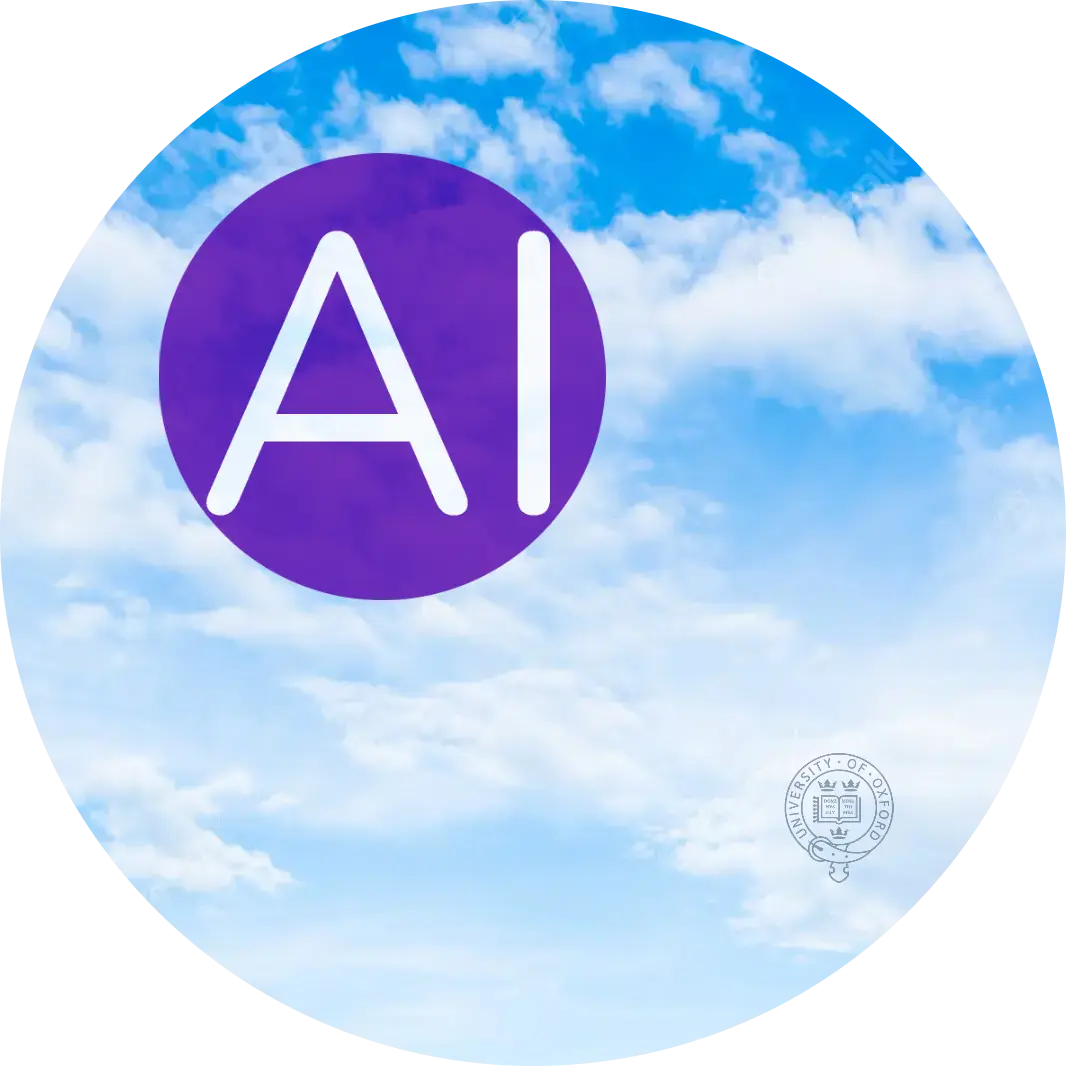