Evidence
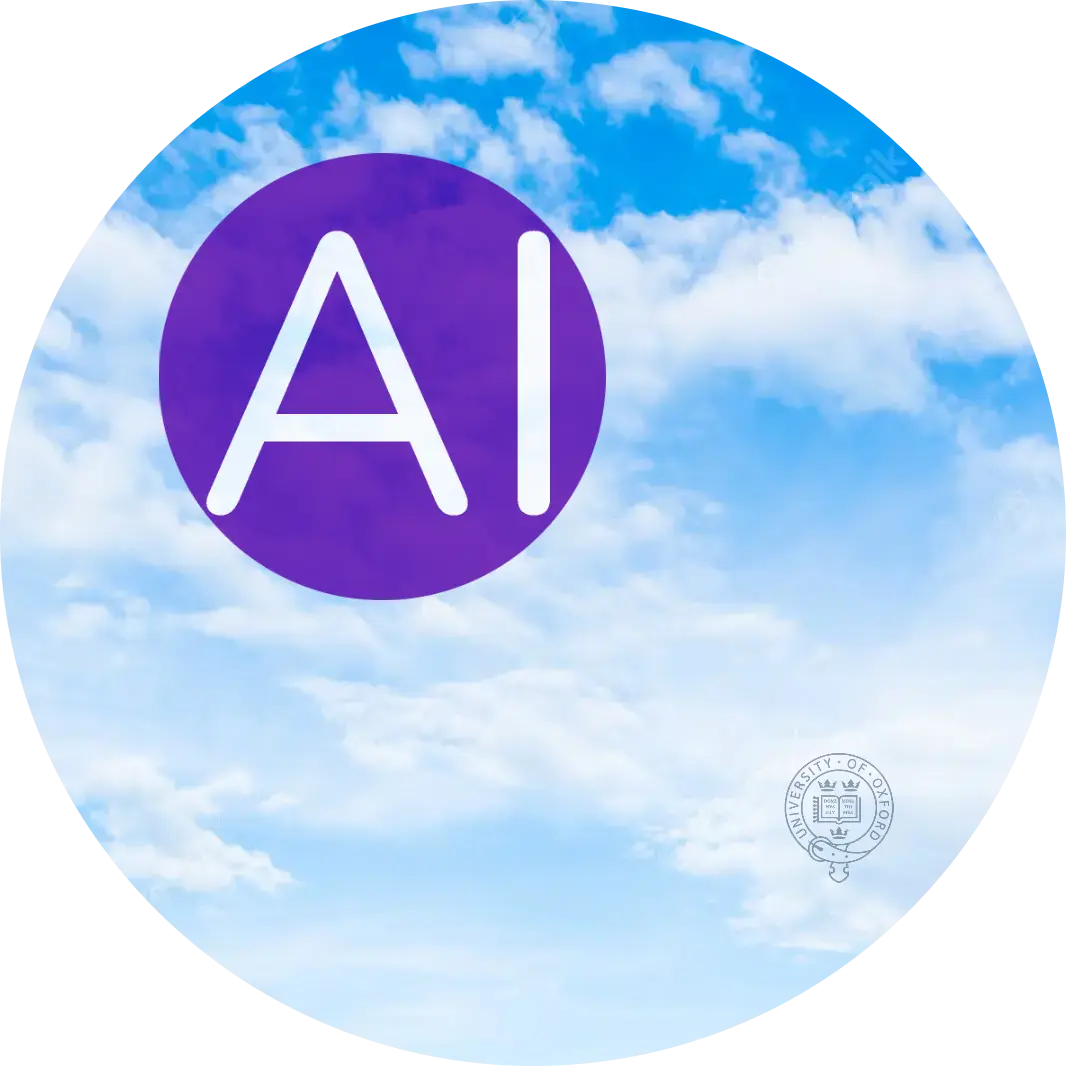
Front Public Health. 2023 Nov 9;11:1271595. doi: 10.3389/fpubh.2023.1271595. eCollection 2023.
ABSTRACT
BACKGROUND: Predicting disability risk in healthy older adults in China is essential for timely preventive interventions, improving their quality of life, and providing scientific evidence for disability prevention. Therefore, developing a machine learning model capable of evaluating disability risk based on longitudinal research data is crucial.
METHODS: We conducted a prospective cohort study of 2,175 older adults enrolled in the China Health and Retirement Longitudinal Study (CHARLS) between 2015 and 2018 to develop and validate this prediction model. Several machine learning algorithms (logistic regression, k-nearest neighbors, naive Bayes, multilayer perceptron, random forest, and XGBoost) were used to assess the 3-year risk of developing disability. The optimal cutoff points and adjustment parameters are explored in the training set, the prediction accuracy of the models is compared in the testing set, and the best-performing models are further interpreted.
RESULTS: During a 3-year follow-up period, a total of 505 (23.22%) healthy older adult individuals developed disabilities. Among the 43 features examined, the LASSO regression identified 11 features as significant for model establishment. When comparing six different machine learning models on the testing set, the XGBoost model demonstrated the best performance across various evaluation metrics, including the highest area under the ROC curve (0.803), accuracy (0.757), sensitivity (0.790), and F1 score (0.789), while its specificity was 0.712. The decision curve analysis (DCA) indicated showed that XGBoost had the highest net benefit in most of the threshold ranges. Based on the importance of features determined by SHAP (model interpretation method), the top five important features were identified as right-hand grip strength, depressive symptoms, marital status, respiratory function, and age. Moreover, the SHAP summary plot was used to illustrate the positive or negative effects attributed to the features influenced by XGBoost. The SHAP dependence plot explained how individual features affected the output of the predictive model.
CONCLUSION: Machine learning-based prediction models can accurately evaluate the likelihood of disability in healthy older adults over a period of 3 years. A combination of XGBoost and SHAP can provide clear explanations for personalized risk prediction and offer a more intuitive understanding of the effect of key features in the model.
PMID:38026309 | PMC:PMC10665855 | DOI:10.3389/fpubh.2023.1271595



Estimated reading time: 6 minute(s)
Latest: Psychiatryai.com #RAISR4D

Cool Evidence: Engaging Young People and Students in Real-World Evidence ☀️

Real-Time Evidence Search [Psychiatry]

AI Research [Andisearch.com]

Disability risk prediction model based on machine learning among Chinese healthy older adults: results from the China Health and Retirement Longitudinal Study
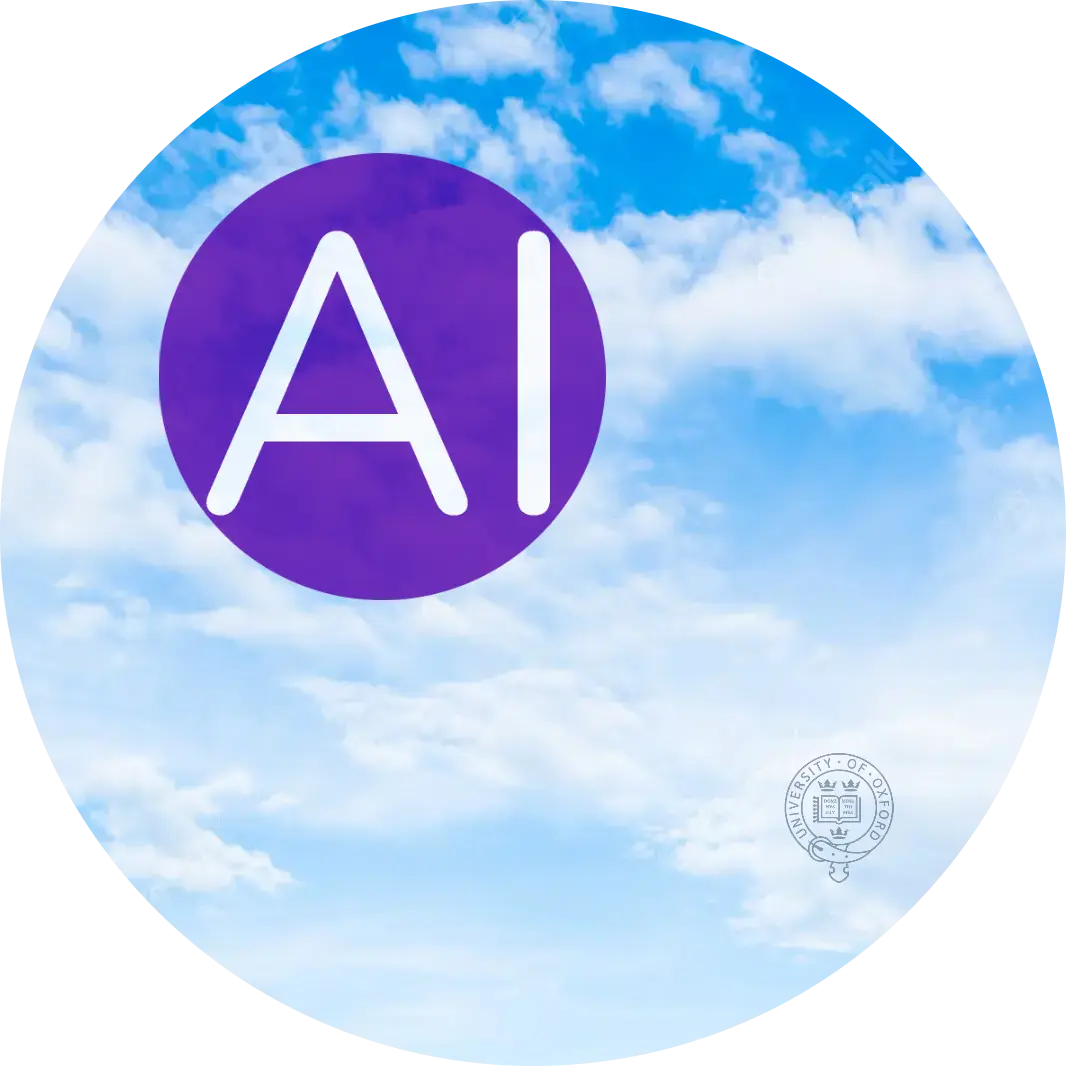
🌐 90 Days
Evidence Blueprint
Disability risk prediction model based on machine learning among Chinese healthy older adults: results from the China Health and Retirement Longitudinal Study
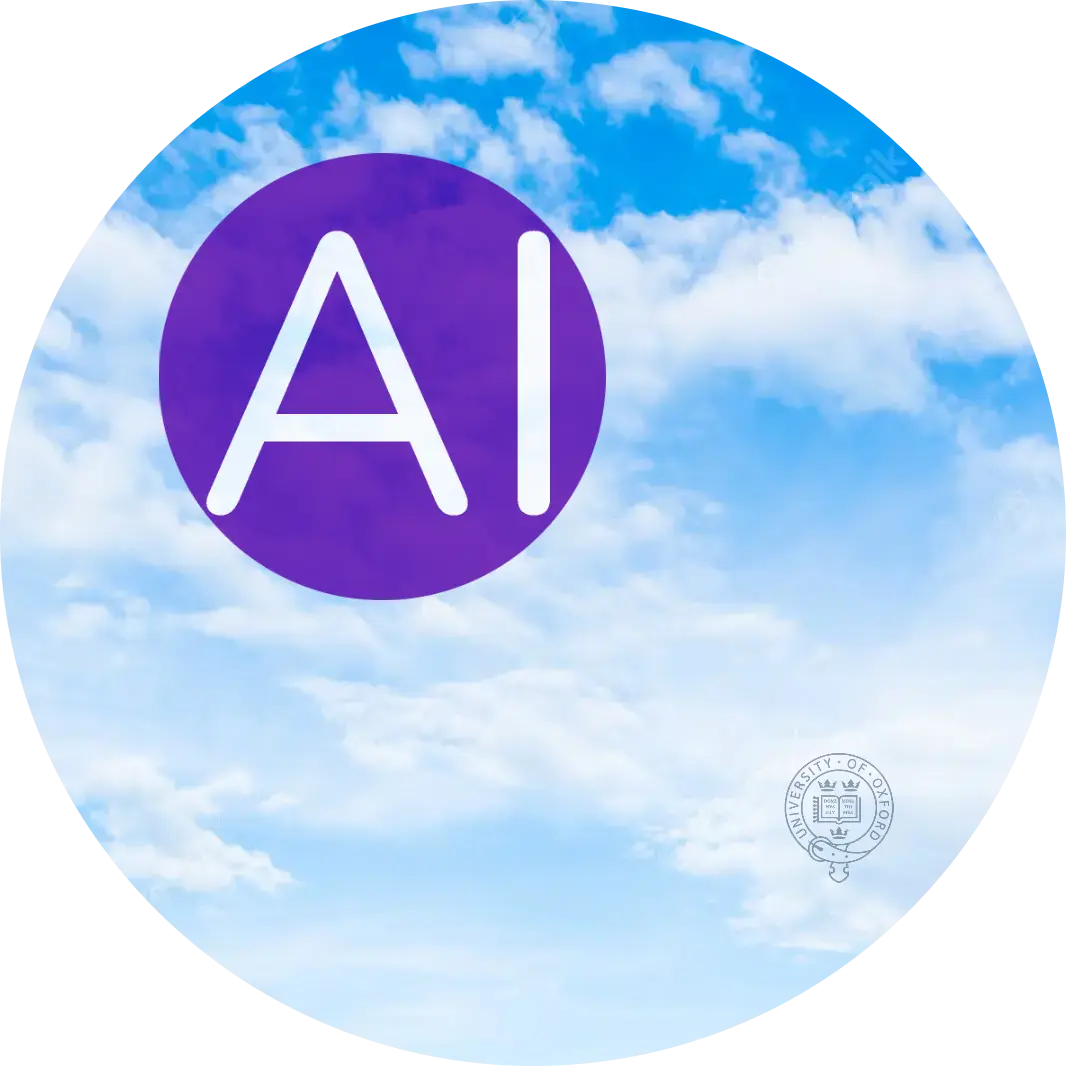
☊ AI-Driven Related Evidence Nodes
(recent articles with at least 5 words in title)
More Evidence
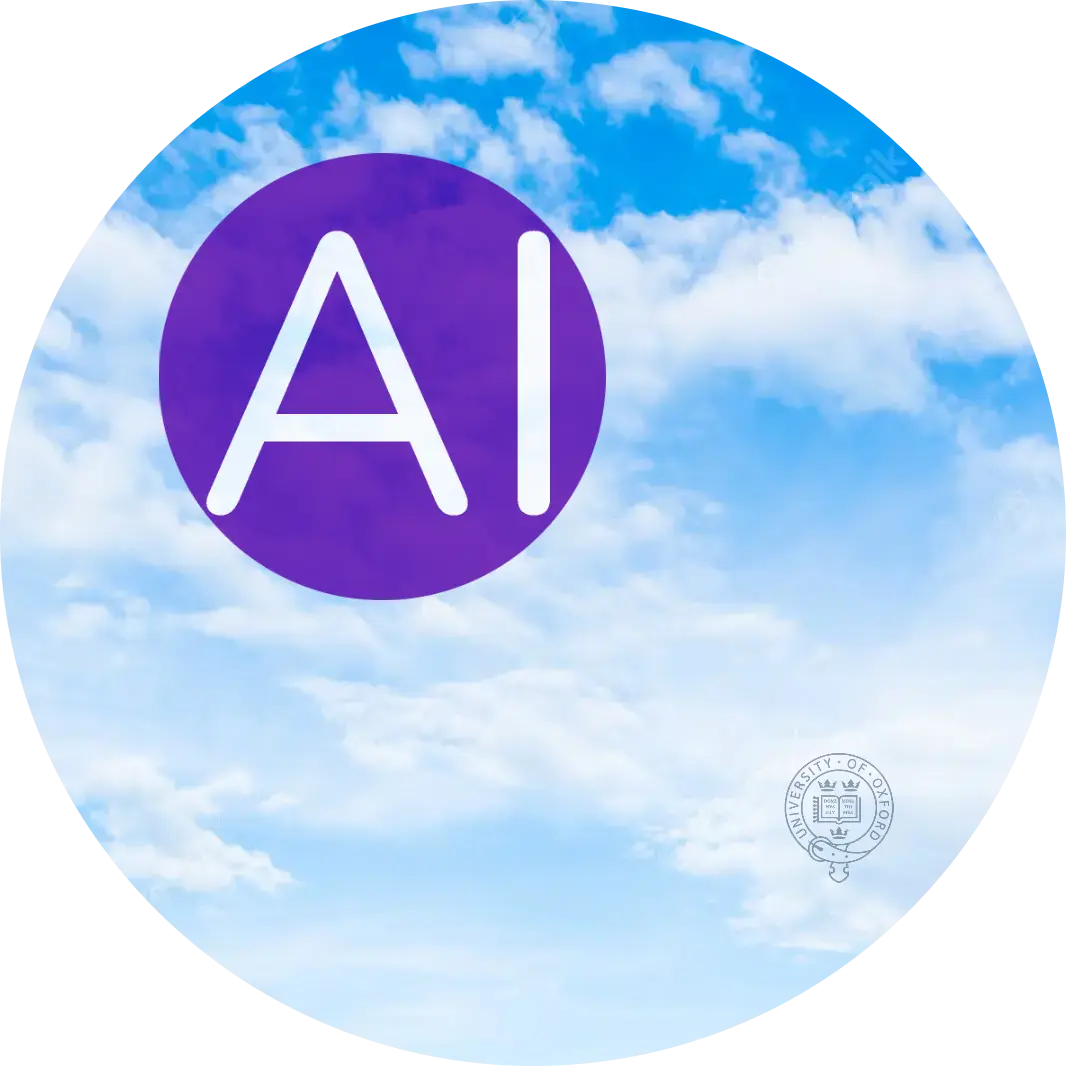