Evidence
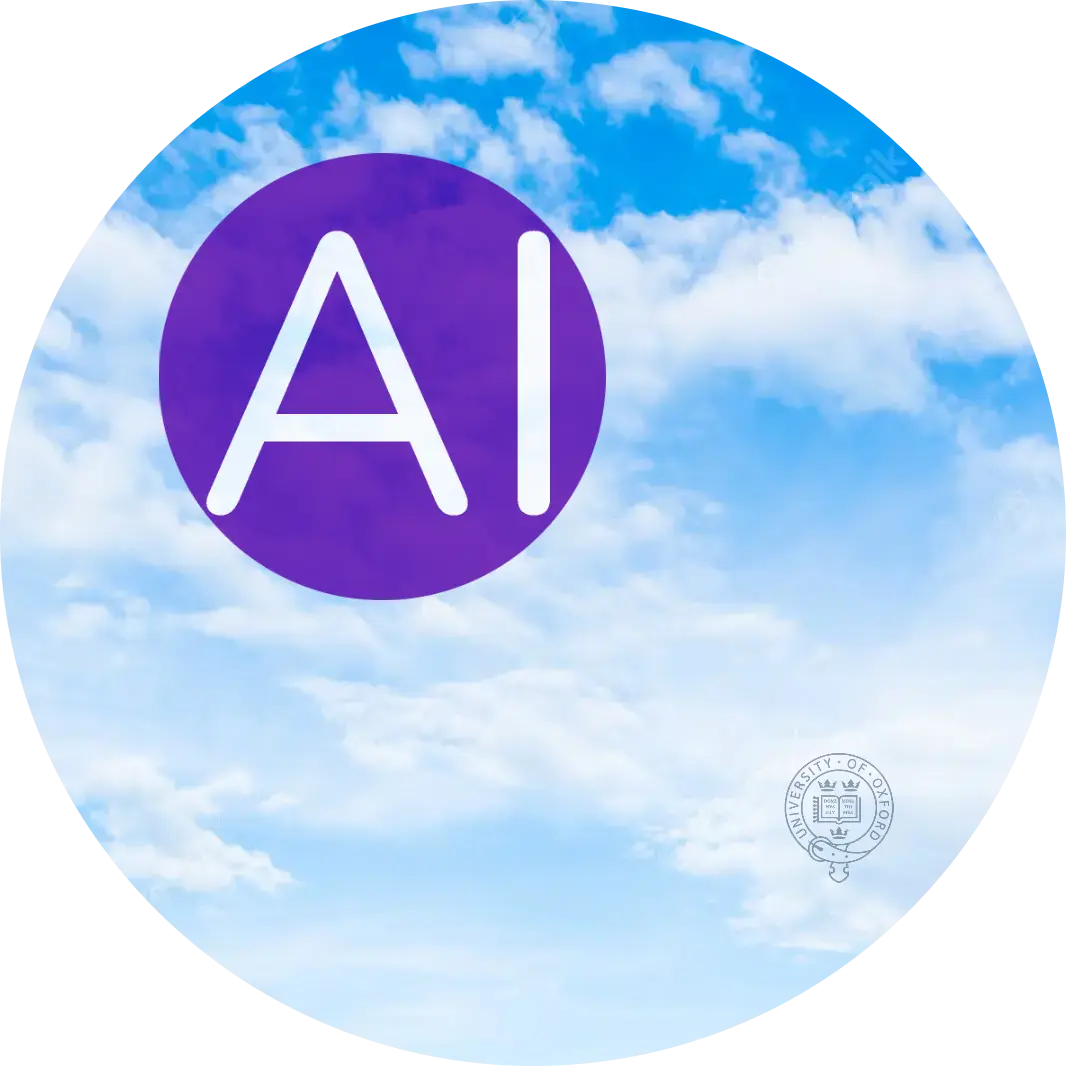
BMC Med Imaging. 2024 May 27;24(1):123. doi: 10.1186/s12880-024-01302-8.
ABSTRACT
The quick proliferation of pandemic diseases has been imposing many concerns on the international health infrastructure. To combat pandemic diseases in smart cities, Artificial Intelligence of Things (AIoT) technology, based on the integration of artificial intelligence (AI) with the Internet of Things (IoT), is commonly used to promote efficient control and diagnosis during the outbreak, thereby minimizing possible losses. However, the presence of multi-source institutional data remains one of the major challenges hindering the practical usage of AIoT solutions for pandemic disease diagnosis. This paper presents a novel framework that utilizes multi-site data fusion to boost the accurateness of pandemic disease diagnosis. In particular, we focus on a case study of COVID-19 lesion segmentation, a crucial task for understanding disease progression and optimizing treatment strategies. In this study, we propose a novel multi-decoder segmentation network for efficient segmentation of infections from cross-domain CT scans in smart cities. The multi-decoder segmentation network leverages data from heterogeneous domains and utilizes strong learning representations to accurately segment infections. Performance evaluation of the multi-decoder segmentation network was conducted on three publicly accessible datasets, demonstrating robust results with an average dice score of 89.9% and an average surface dice of 86.87%. To address scalability and latency issues associated with centralized cloud systems, fog computing (FC) emerges as a viable solution. FC brings resources closer to the operator, offering low latency and energy-efficient data management and processing. In this context, we propose a unique FC technique called PANDFOG to deploy the multi-decoder segmentation network on edge nodes for practical and clinical applications of automated COVID-19 pneumonia analysis. The results of this study highlight the efficacy of the multi-decoder segmentation network in accurately segmenting infections from cross-domain CT scans. Moreover, the proposed PANDFOG system demonstrates the practical deployment of the multi-decoder segmentation network on edge nodes, providing real-time access to COVID-19 segmentation findings for improved patient monitoring and clinical decision-making.
PMID:38797827 | DOI:10.1186/s12880-024-01302-8



Estimated reading time: 6 minute(s)
Latest: Psychiatryai.com #RAISR4D Evidence

Cool Evidence: Engaging Young People and Students in Real-World Evidence

Real-Time Evidence Search [Psychiatry]

AI Research

Fog-based deep learning framework for real-time pandemic screening in smart cities from multi-site tomographies
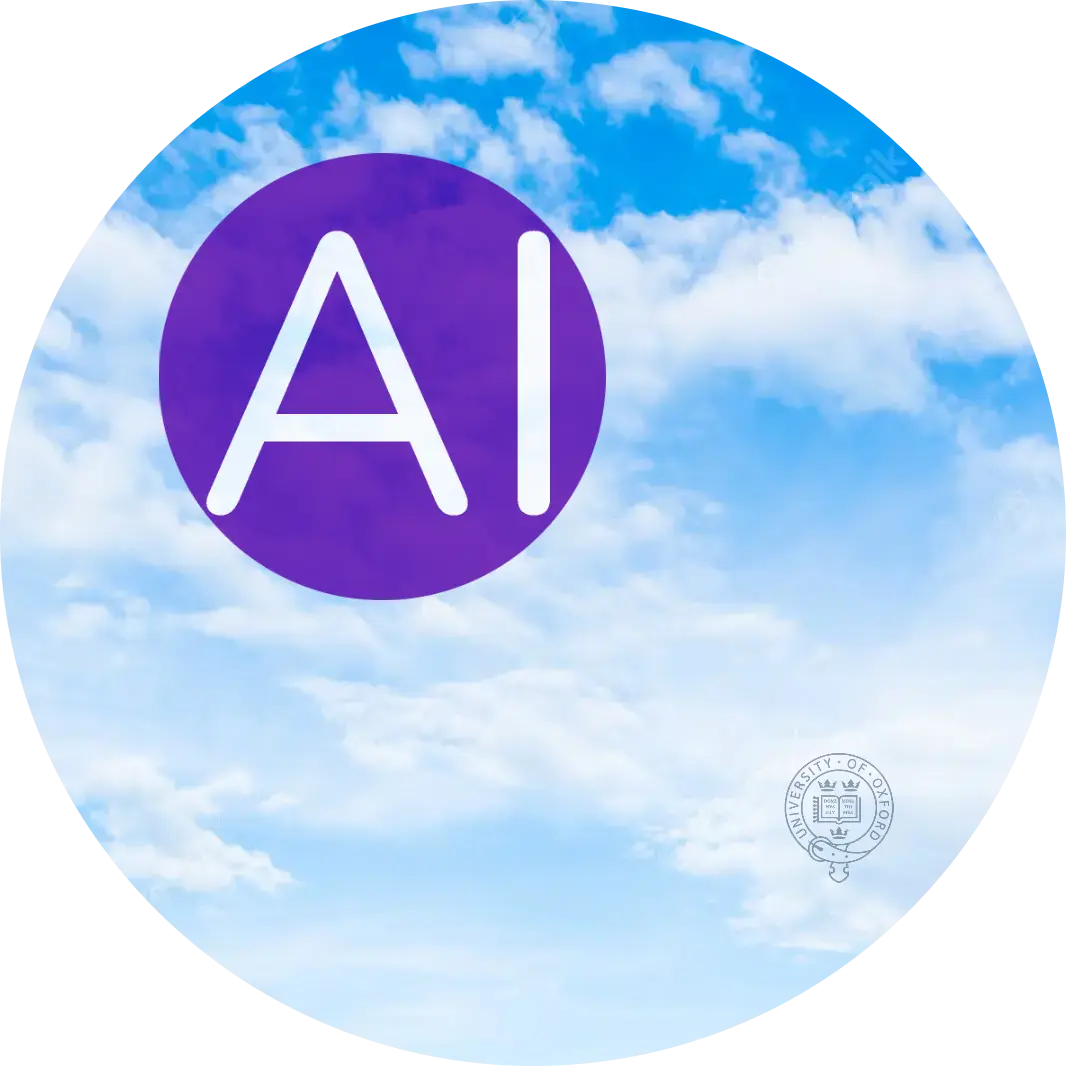
🌐 90 Days
Evidence Blueprint
Fog-based deep learning framework for real-time pandemic screening in smart cities from multi-site tomographies
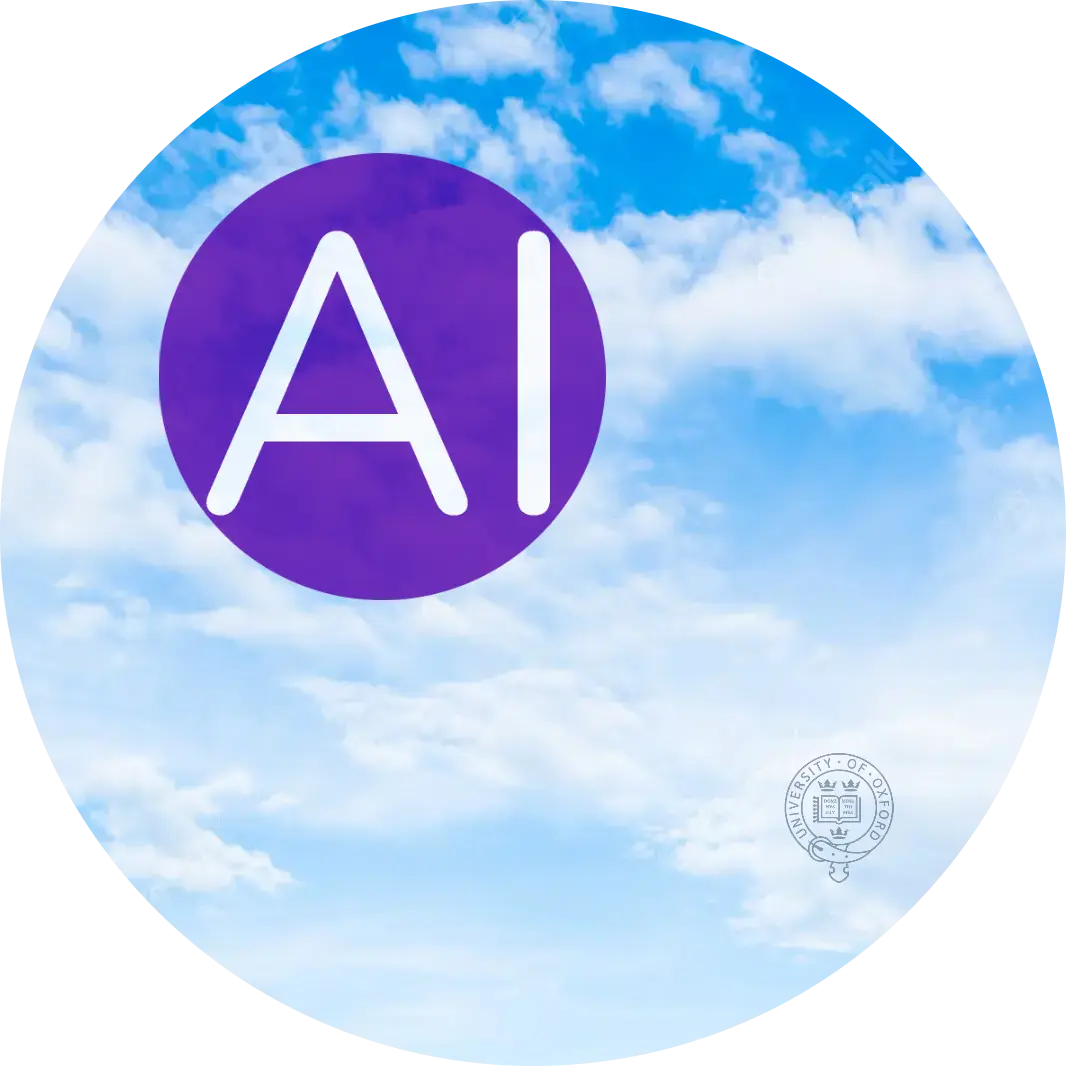
☊ AI-Driven Related Evidence Nodes
(recent articles with at least 5 words in title)
More Evidence
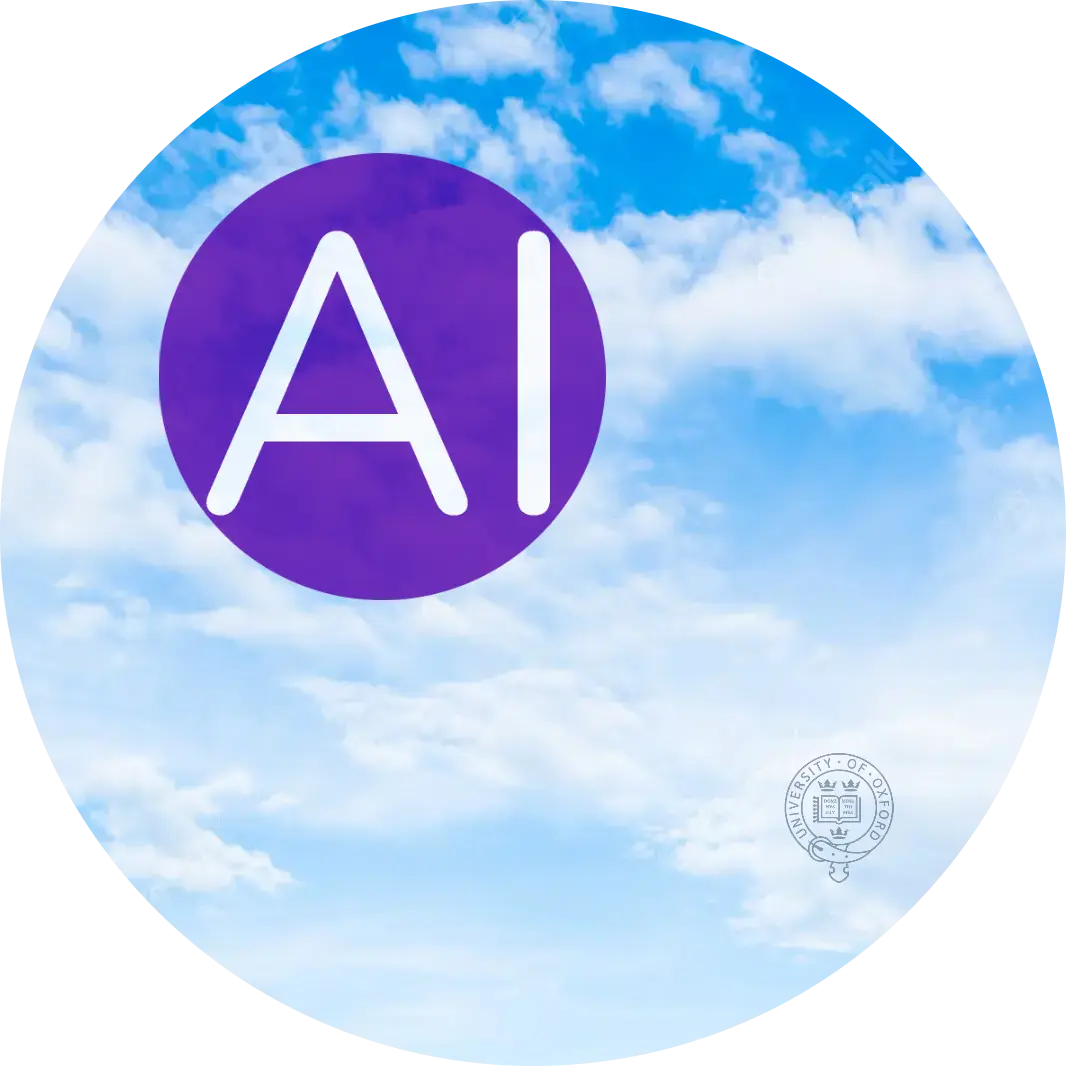