Evidence
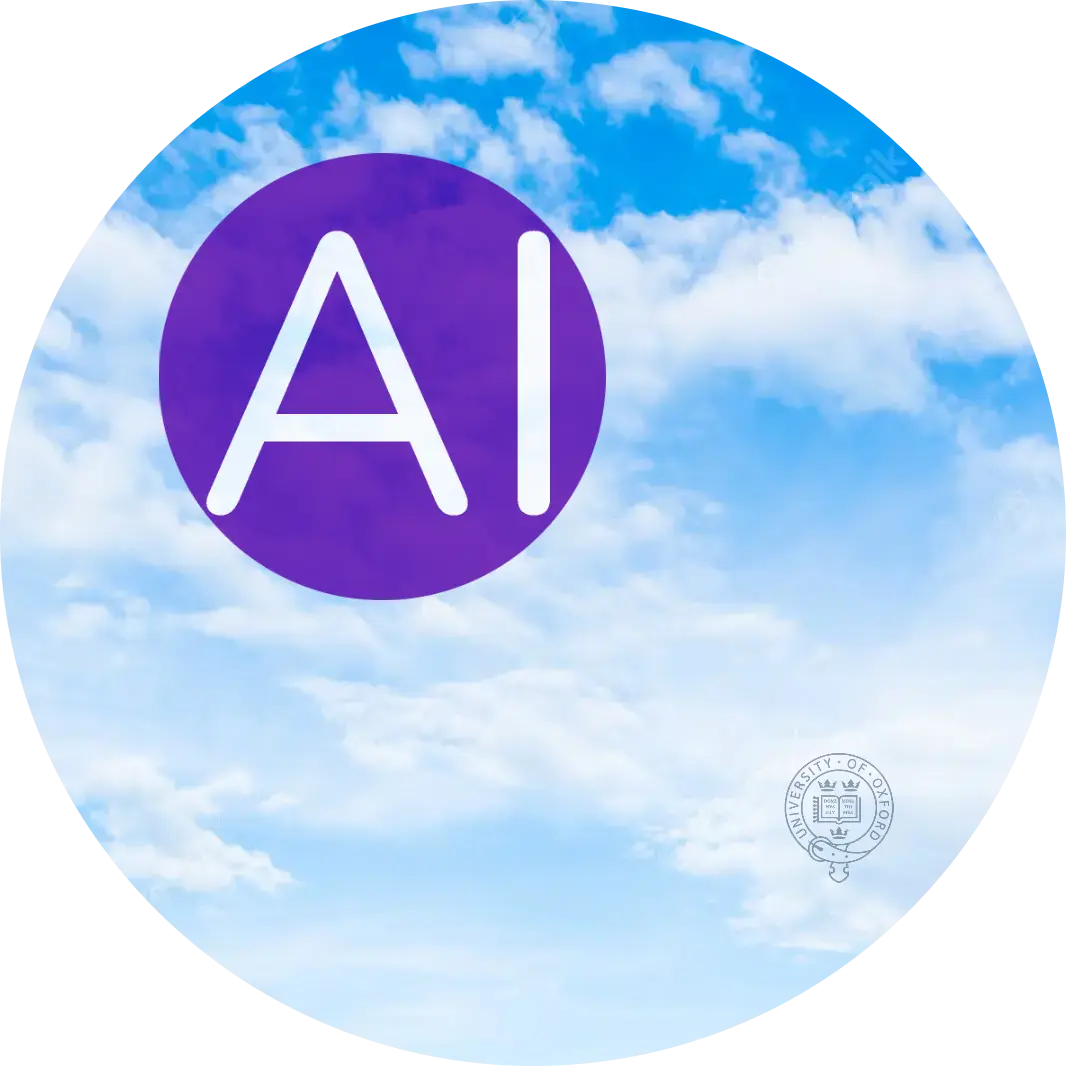
Heart Rhythm. 2024 Aug 19:S1547-5271(24)03149-7. doi: 10.1016/j.hrthm.2024.08.030. Online ahead of print.
ABSTRACT
BACKGROUND: Arrhythmogenic right ventricular cardiomyopathy (ARVC) is a rare genetic heart disease associated with life-threatening ventricular arrhythmias. Diagnosis of ARVC is based on the 2010 Task Force Criteria (TFC), application of which often requires clinical expertise at specialized centers.
OBJECTIVE: To develop and validate an electrocardiogram (ECG) deep-learning (DL) tool for ARVC diagnosis.
METHODS: ECGs of patients referred for ARVC evaluation were used to develop (n=551, 80.1%) and test (n=137, 19.9%) an ECG-DL model for prediction of TFC-defined ARVC diagnosis. The ARVC ECG-DL model was externally validated in a cohort of patients with pathogenic or likely pathogenic (P/LP) ARVC gene variants identified through the Geisinger MyCode Community Health Initiative (N=167).
RESULTS: Of 688 patients evaluated at JHH (57.3% male, mean age 40.2 years), 329 (47.8%) were diagnosed with ARVC. While ARVC diagnosis made by referring cardiologist ECG interpretation was unreliable (c-statistic 0.53 [CI: 0.52, 0.53]), ECG-DL discrimination in the hold-out testing cohort was excellent (0.87 [0.86, 0.89]) and compared favorably to that of ECG interpretation by an ARVC expert (0.85 [0.84, 0.86]). In the Geisinger cohort, prevalence of ARVC was lower (n=17, 10.2%), but ECG-DL based identification of ARVC phenotype remained reliable (0.80 [0.77, 0.83]). Discrimination was further increased when ECG-DL predictions were combined with non-ECG-derived TFC in the JHH testing (c-statistic 0.940 [95%CI: 0.933; 0.948]) and Geisinger validation (0.897 [95%CI: 0.883; 0.912]) cohorts.
CONCLUSION: ECG-DL augments diagnosis of ARVC to the level of an ARVC expert and can differentiate true ARVC diagnosis from phenotype-mimics and at-risk family members/genotype-positive individuals.
PMID:39168295 | DOI:10.1016/j.hrthm.2024.08.030



Estimated reading time: 5 minute(s)
Latest: Psychiatryai.com #RAISR4D Evidence

Cool Evidence: Engaging Young People and Students in Real-World Evidence

Real-Time Evidence Search [Psychiatry]

AI Research

Improved diagnosis of arrhythmogenic right ventricular cardiomyopathy using electrocardiographic deep-learning
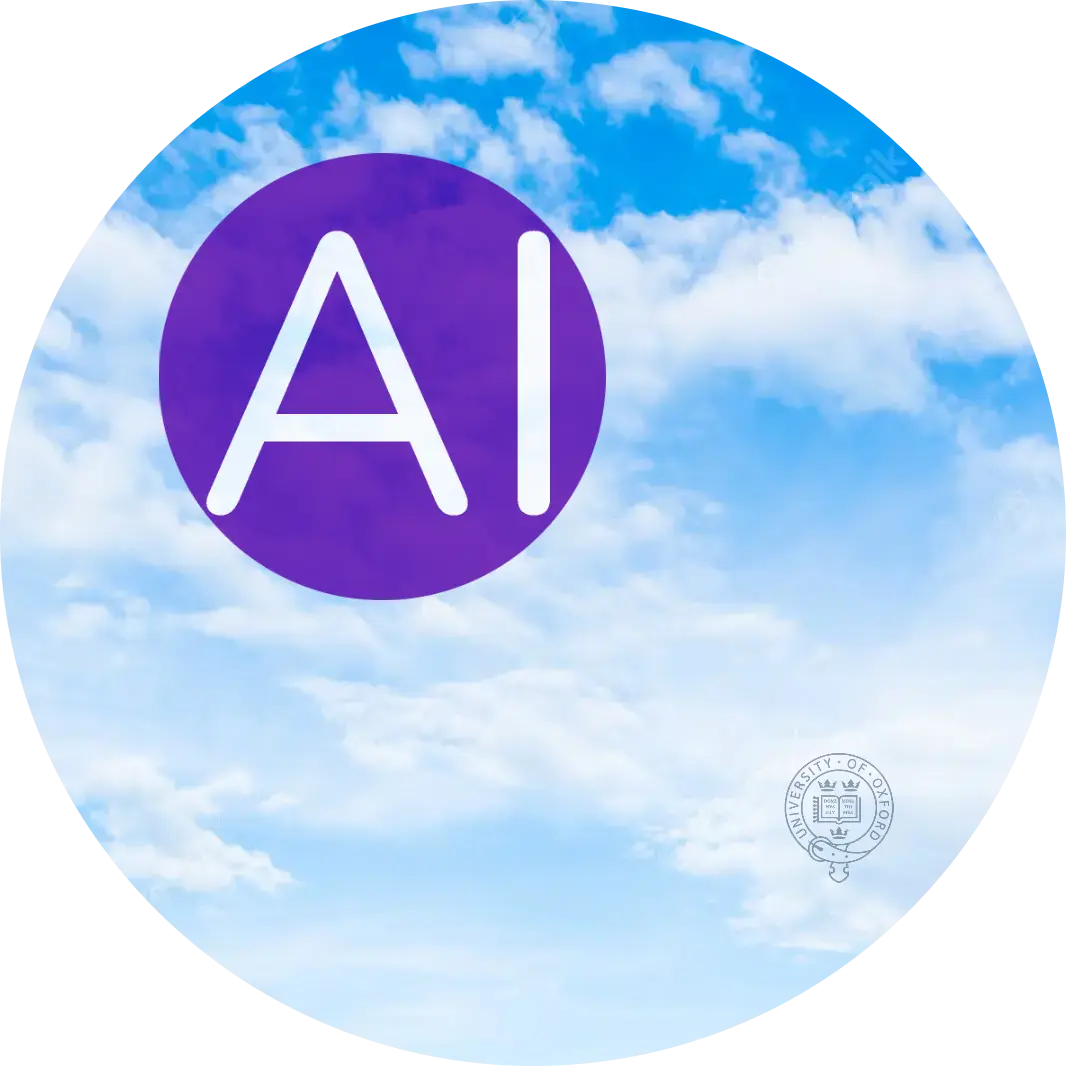
🌐 90 Days
Evidence Blueprint
Improved diagnosis of arrhythmogenic right ventricular cardiomyopathy using electrocardiographic deep-learning
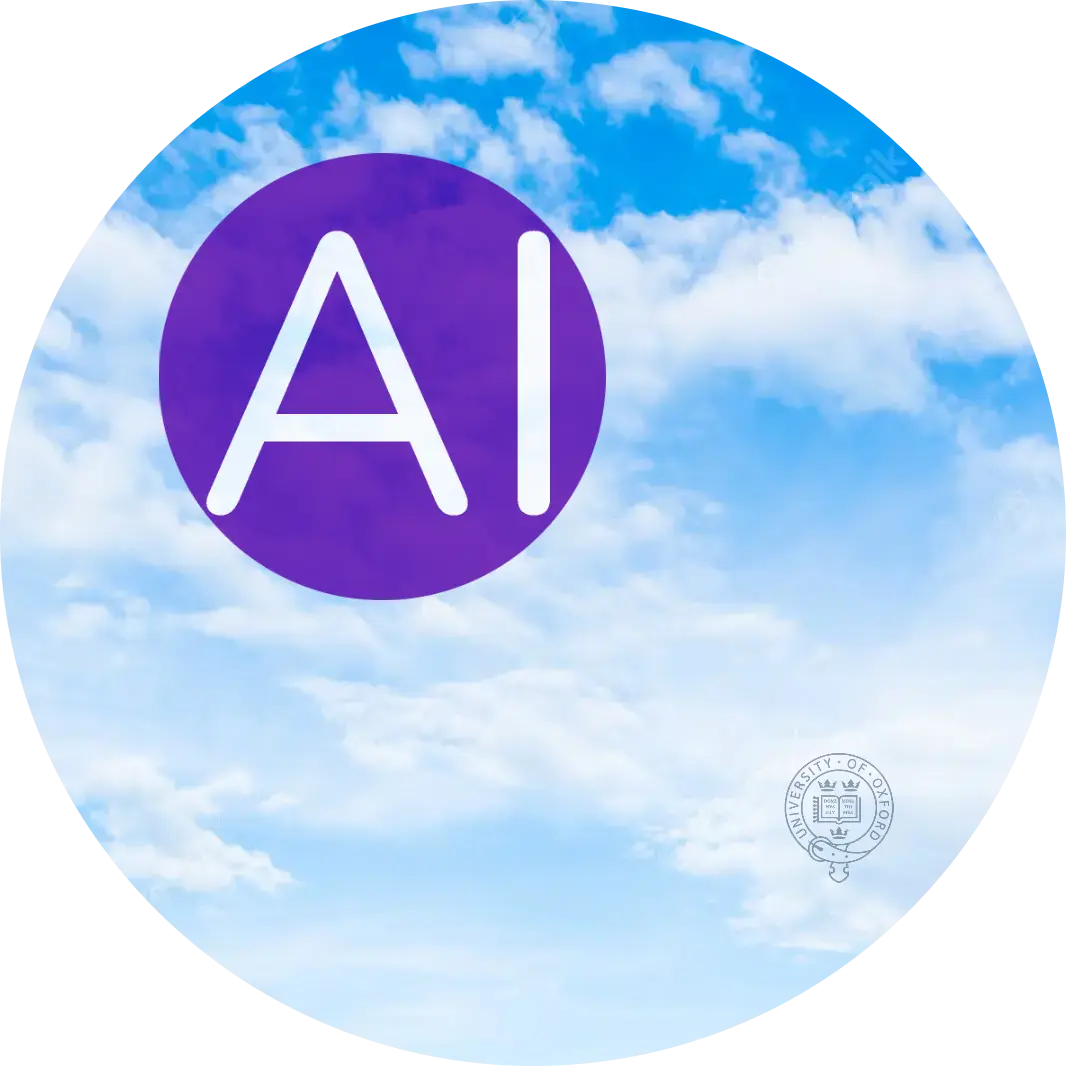
☊ AI-Driven Related Evidence Nodes
(recent articles with at least 5 words in title)
More Evidence
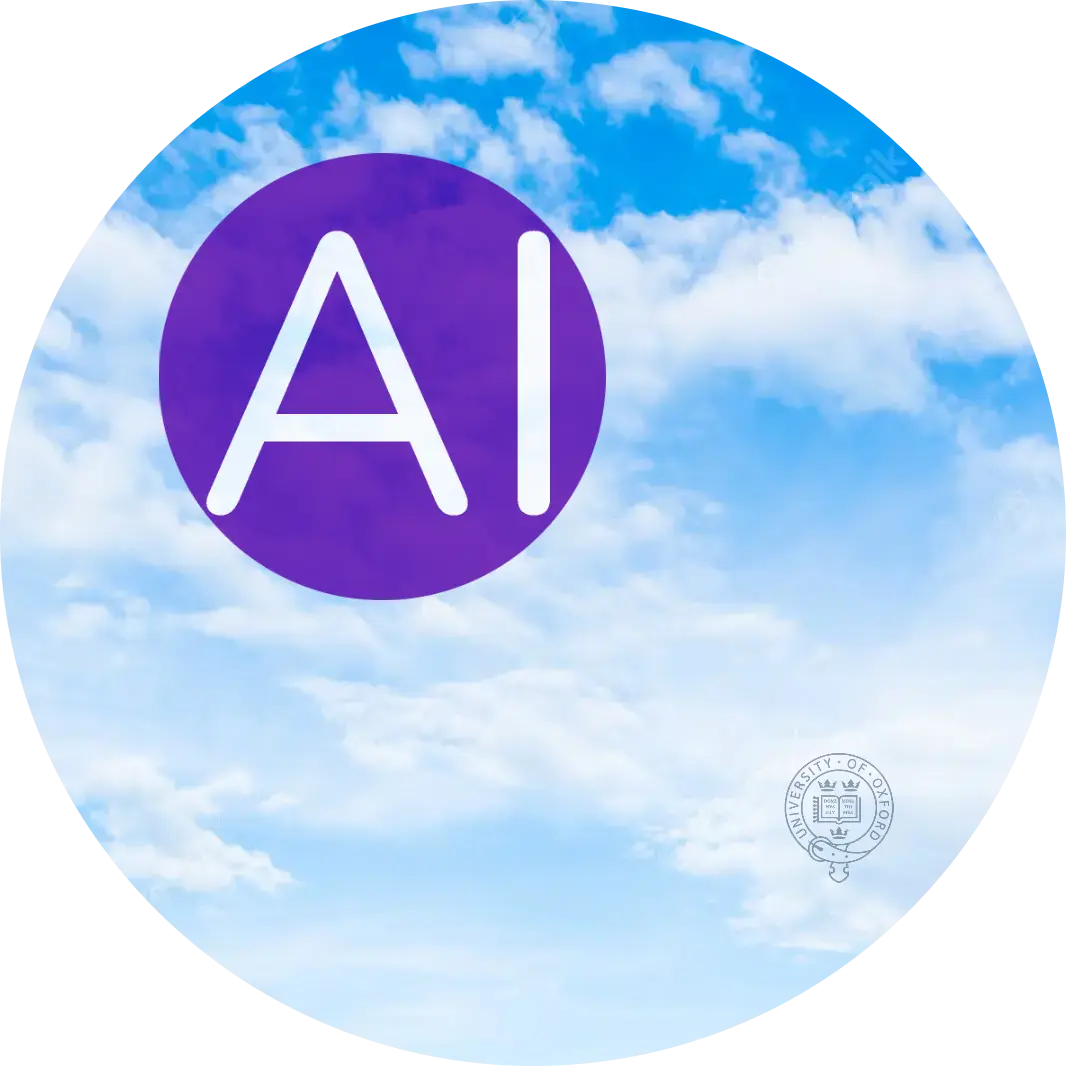