Evidence
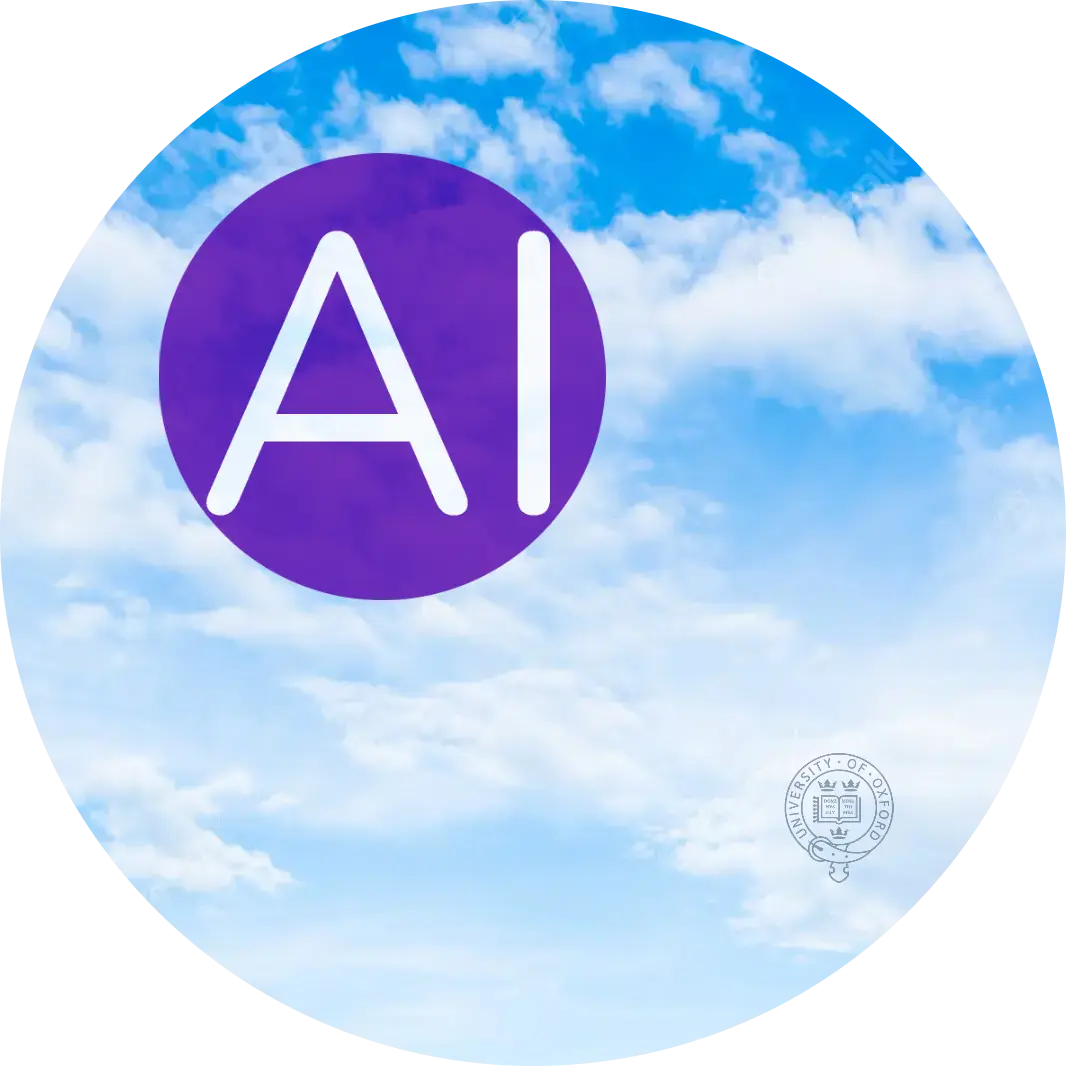
ACS Appl Mater Interfaces. 2024 Mar 21. doi: 10.1021/acsami.4c00752. Online ahead of print.
ABSTRACT
Significant progress has been made in designing advanced membranes; however, persistent challenges remain due to their reduced permeation rates and a propensity for substantial fouling. These factors continue to pose significant barriers to the effective utilization of membranes in the separation of oil-in-water emulsions. Metal-organic frameworks (MOFs) are considered promising materials for such applications; however, they encounter three key challenges when applied to the separation of oil from water: (a) lack of water stability; (b) difficulty in producing defect-free membranes; and (c) unresolved issue of stabilizing the MOF separating layer on the ceramic membrane (CM) support. In this study, a defect-free hydrolytically stable zirconium-based MOF separating layer was formed through a two-step method: first, by in situ growth of UiO-66-NH2 MOF into the voids of polydopamine (PDA)-functionalized CM during the solvothermal process, and then by facilitating the self-assembly of UiO-66-NH2 with PDA using a pressurized dead-end assembly. A stable MOF separating layer was attained by enriching the ceramic support with amines and hydroxyl groups using PDA, which assisted in the assembly and stabilization of UiO-66-NH2. The PDA-s-UiO-66-NH2-CM membrane displayed air superhydrophilicity and underwater superoleophobicity, demonstrating its oil resistance and high antifouling behavior. The PDA-s-UiO-66-NH2-CM membrane has shown exceptionally high permeability and separation capacity for challenging oil-in-water emulsions. This is attributed to numerous nanochannels from the membrane and its high resistance to oil adhesion. The membranes showed excellent stability over 15 continuous test cycles, which indicates that the developed MOFs separating layers have a low tendency to be clogged by oil droplets during separation. Machine learning-based Gaussian process regression (GPR) models as nonparametric kernel-based probabilistic models were employed to predict the performance efficiency of the PDA-s-UiO-66-NH2-CM membrane in oil-in-water separation. The outcomes were compared with the support vector machine (SVM) and decision tree (DT) algorithm. This efficiency includes various metrics related to its separation accuracy, and the models were developed through feature engineering to identify and utilize the most significant factors affecting the membrane’s performance. The results proved the reliability of GPR optimization with the highest prediction accuracy in the validation phase. The average percentage increase of the GPR model compared to the SVM and DT model was 6.11 and 42.94%, respectively.
PMID:38514254 | DOI:10.1021/acsami.4c00752



Estimated reading time: 7 minute(s)
Latest: Psychiatryai.com #RAISR4D
Real-Time Evidence Search [Psychiatry]
Design and Machine Learning Prediction of In Situ Grown PDA-Stabilized MOF (UiO-66-NH2) Membrane for Low-Pressure Separation of Emulsified Oily Wastewater
🌐 90 Days
Evidence Blueprint
Design and Machine Learning Prediction of In Situ Grown PDA-Stabilized MOF (UiO-66-NH2) Membrane for Low-Pressure Separation of Emulsified Oily Wastewater
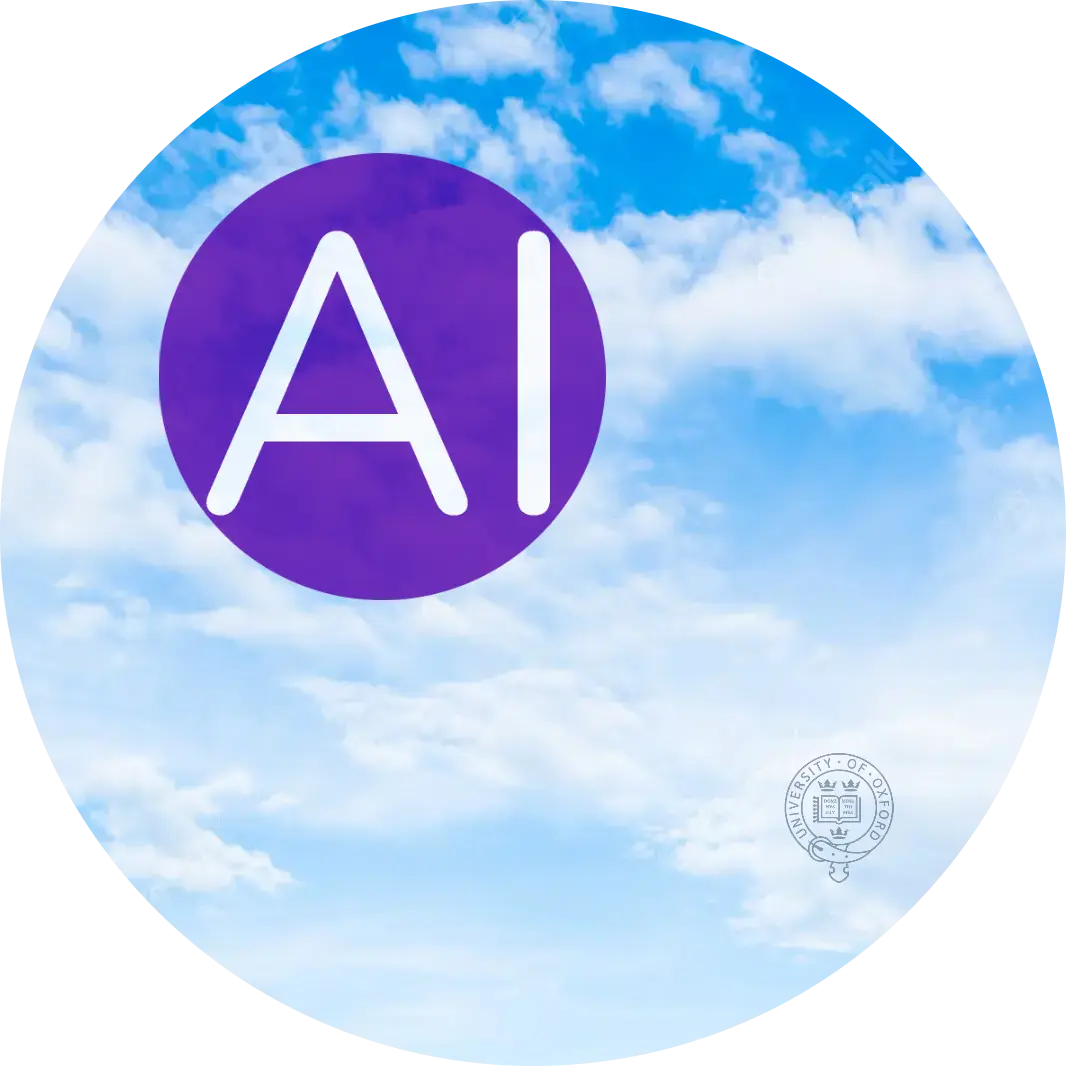
☊ AI-Driven Related Evidence Nodes
(recent articles with at least 5 words in title)
Save Evidence Blueprint
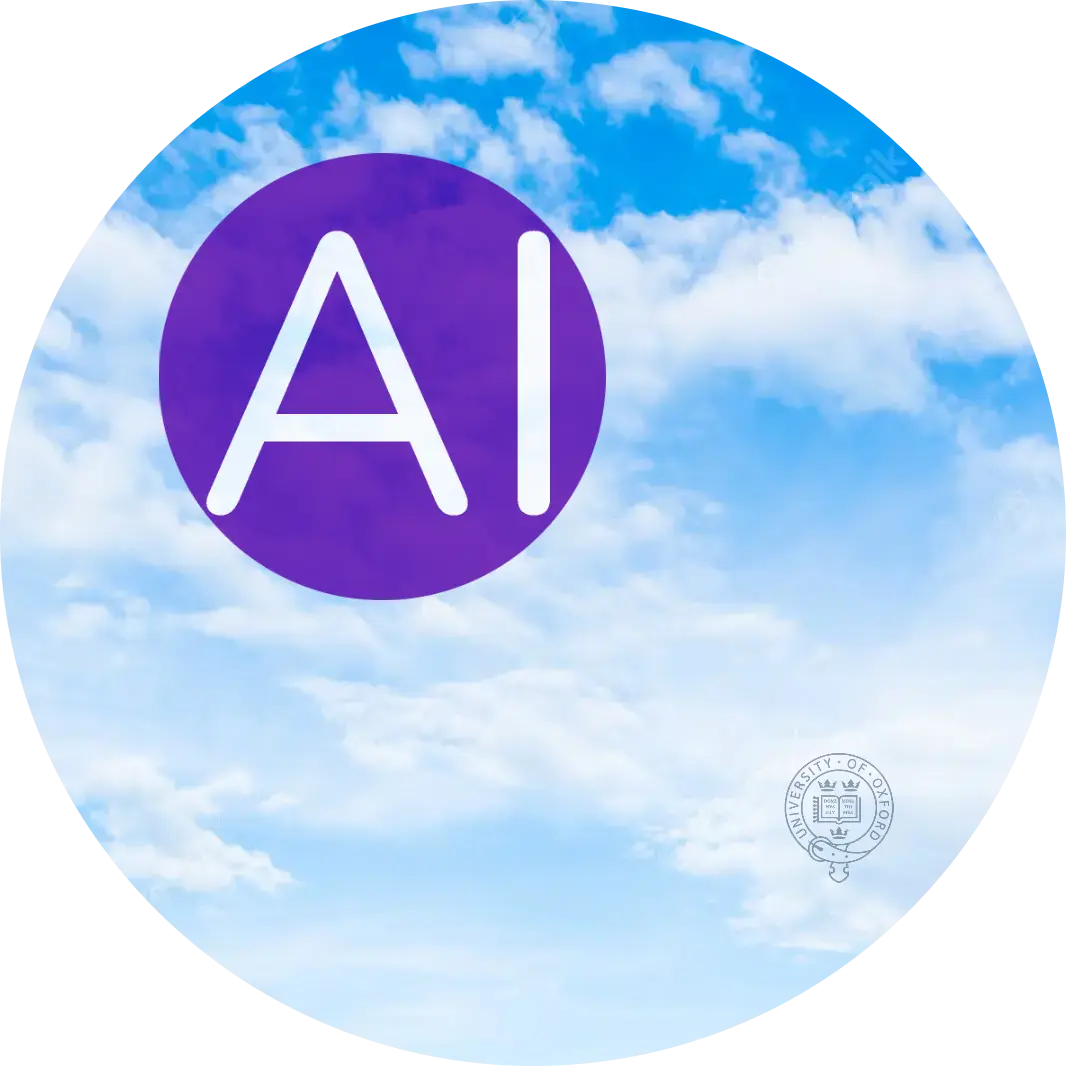
Save as PDF