Evidence
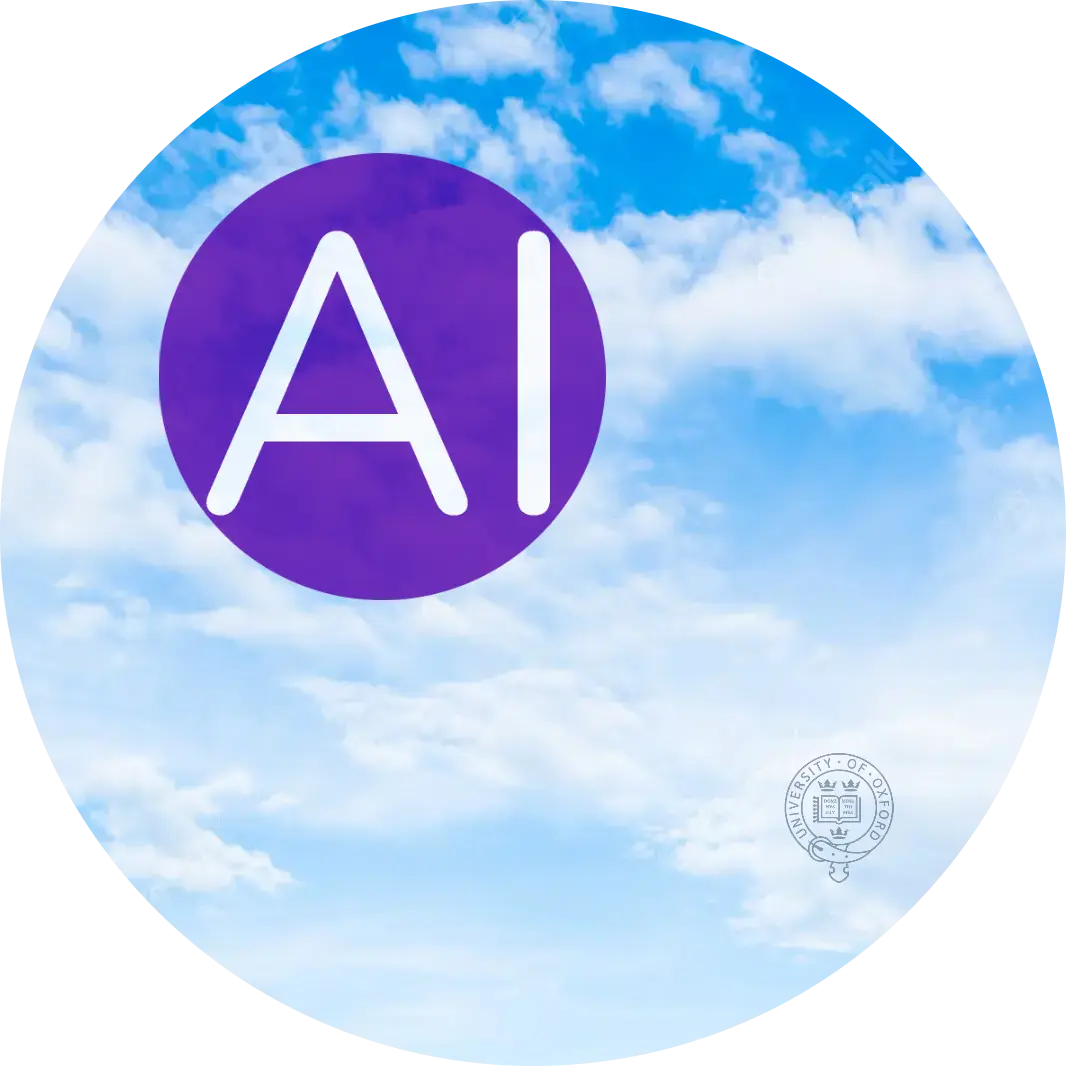
IEEE Trans Neural Netw Learn Syst. 2023 Jun 20;PP. doi: 10.1109/TNNLS.2023.3282961. Online ahead of print.
ABSTRACT
Functional connectivity network (FCN) data from functional magnetic resonance imaging (fMRI) is increasingly used for the diagnosis of brain disorders. However, state-of-the-art studies used to build the FCN using a single brain parcellation atlas at a certain spatial scale, which largely neglected functional interactions across different spatial scales in hierarchical manners. In this study, we propose a novel framework to perform multiscale FCN analysis for brain disorder diagnosis. We first use a set of well-defined multiscale atlases to compute multiscale FCNs. Then, we utilize biologically meaningful brain hierarchical relationships among the regions in multiscale atlases to perform nodal pooling across multiple spatial scales, namely “Atlas-guided Pooling (AP).” Accordingly, we propose a multiscale-atlases-based hierarchical graph convolutional network (MAHGCN), built on the stacked layers of graph convolution and the AP, for a comprehensive extraction of diagnostic information from multiscale FCNs. Experiments on neuroimaging data from 1792 subjects demonstrate the effectiveness of our proposed method in the diagnoses of Alzheimer’s disease (AD), the prodromal stage of AD i.e., mild cognitive impairment (MCI), as well as autism spectrum disorder (ASD), with the accuracy of 88.9%, 78.6%, and 72.7%, respectively. All results show significant advantages of our proposed method over other competing methods. This study not only demonstrates the feasibility of brain disorder diagnosis using resting-state fMRI empowered by deep learning but also highlights that the functional interactions in the multiscale brain hierarchy are worth being explored and integrated into deep learning network architectures for a better understanding of the neuropathology of brain disorders. The codes for MAHGCN are publicly available at “https://github.com/MianxinLiu/ MAHGCN-code.”
PMID:37339027 | DOI:10.1109/TNNLS.2023.3282961



Estimated reading time: 5 minute(s)
Latest: Psychiatryai.com #RAISR4D

Cool Evidence: Engaging Young People and Students in Real-World Evidence ☀️

Real-Time Evidence Search [Psychiatry]

AI Research [Andisearch.com]

Hierarchical Graph Convolutional Network Built by Multiscale Atlases for Brain Disorder Diagnosis Using Functional Connectivity
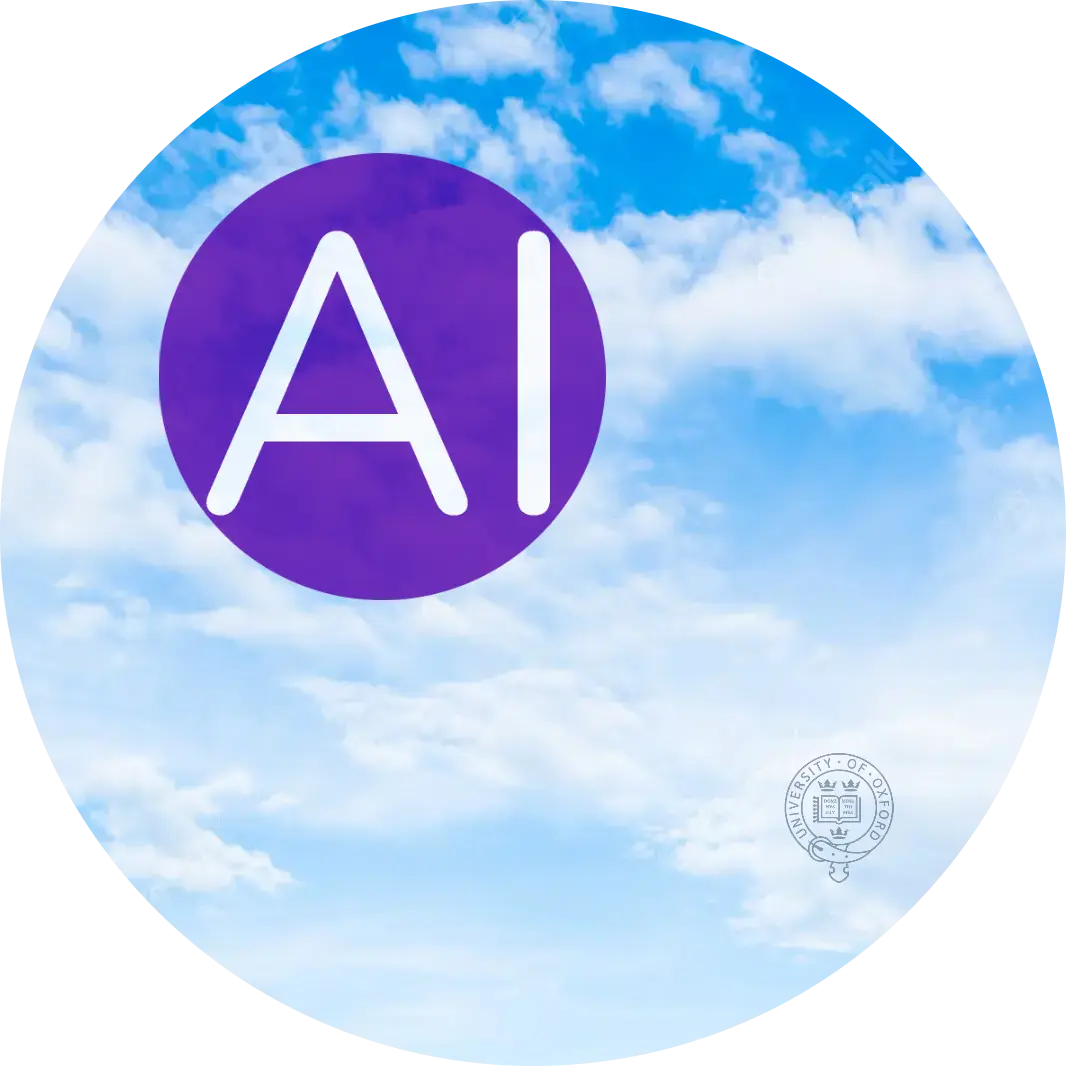
🌐 90 Days
Evidence Blueprint
Hierarchical Graph Convolutional Network Built by Multiscale Atlases for Brain Disorder Diagnosis Using Functional Connectivity
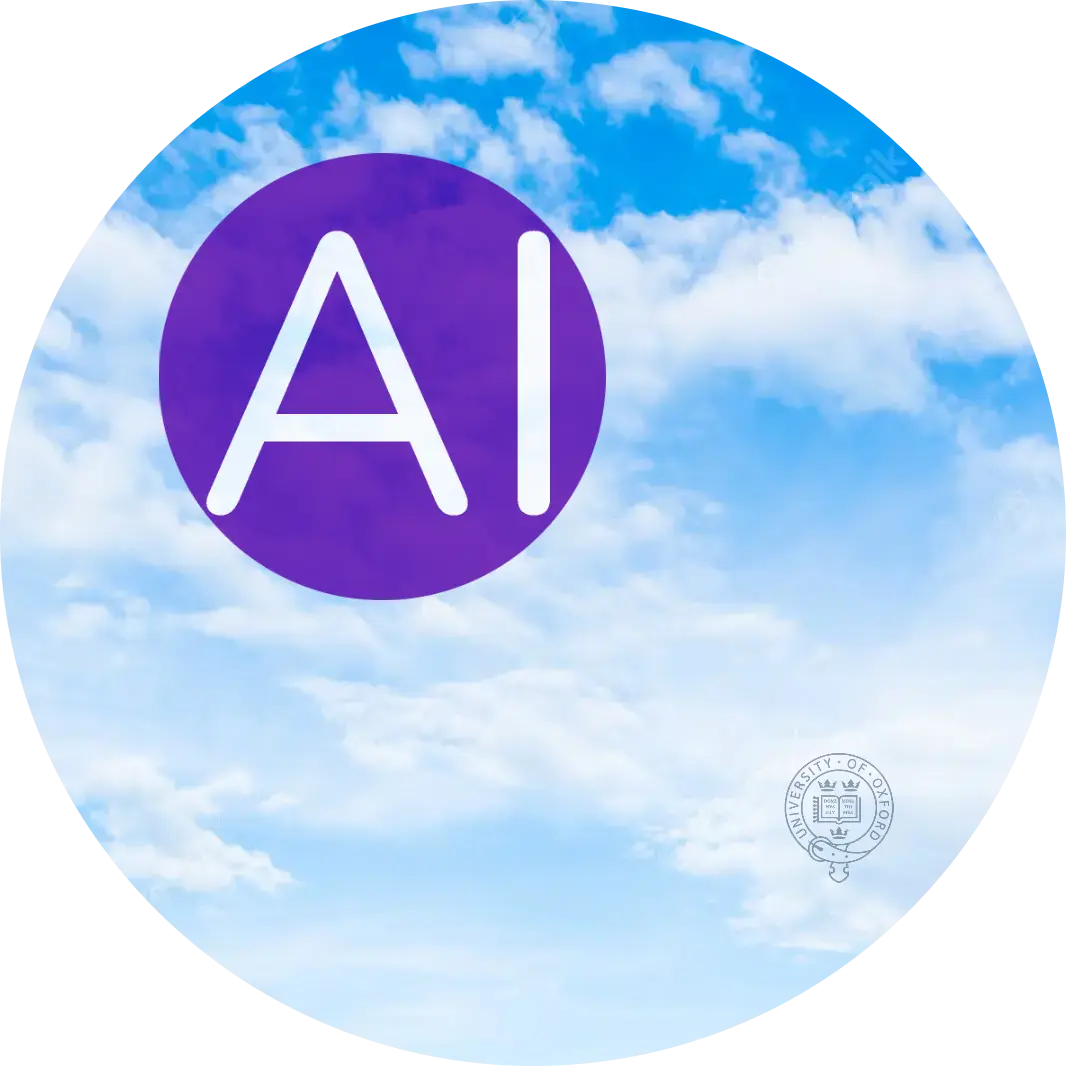
☊ AI-Driven Related Evidence Nodes
(recent articles with at least 5 words in title)
More Evidence
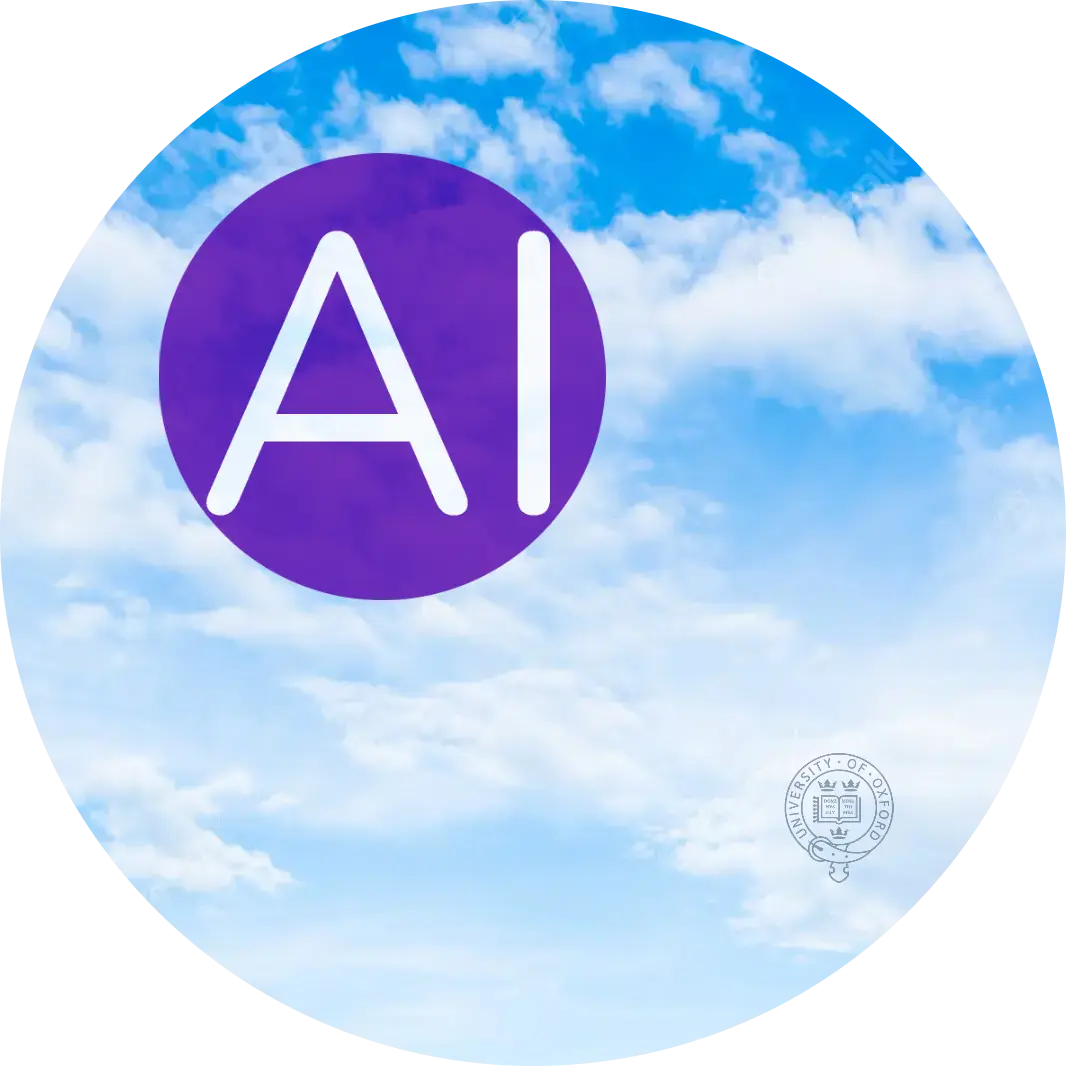