Evidence
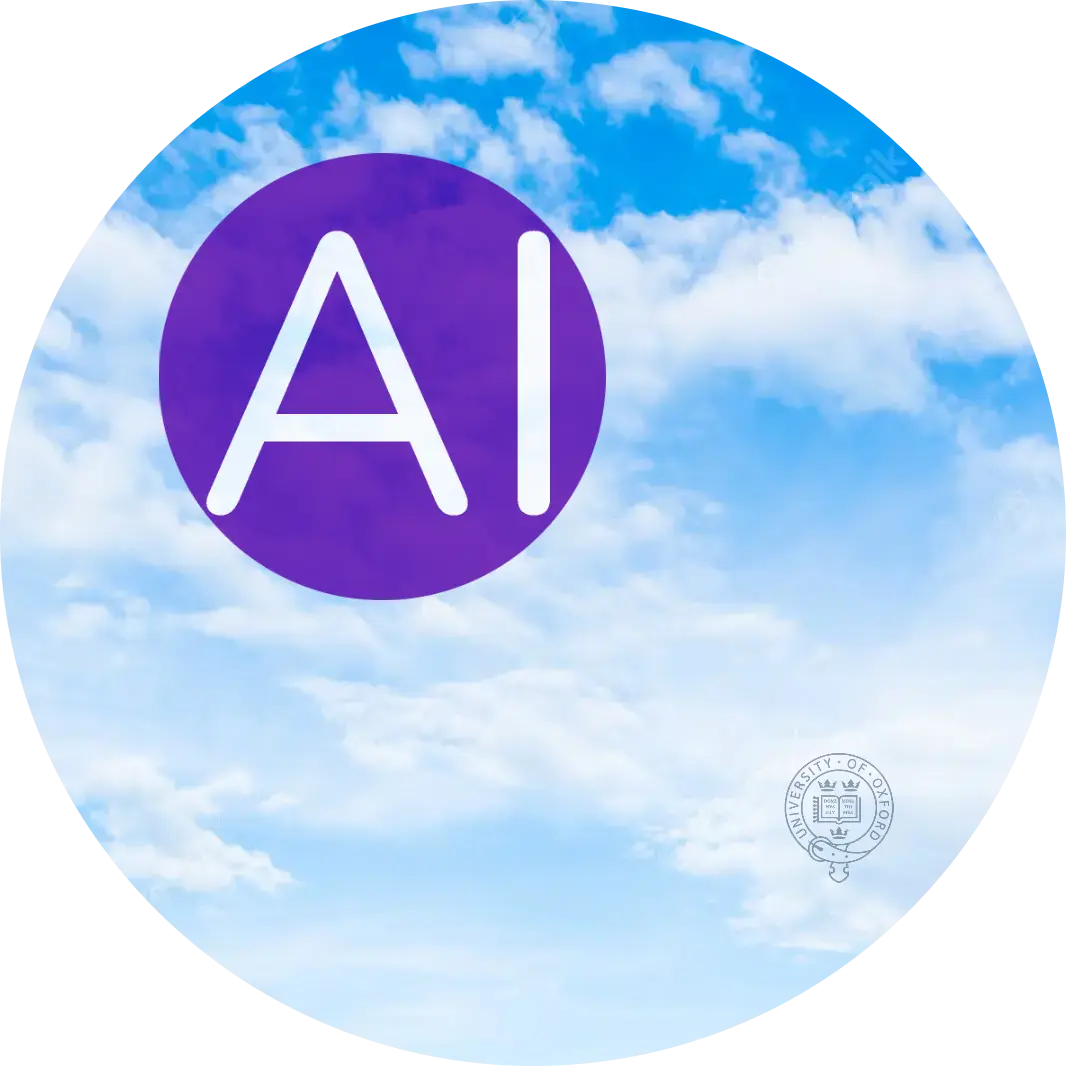
Neuroinformatics. 2023 Aug 15. doi: 10.1007/s12021-023-09639-1. Online ahead of print.
ABSTRACT
Resting-state functional magnetic resonance imaging (rs-fMRI) is a non-invasive imaging technique widely used in neuroscience to understand the functional connectivity of the human brain. While rs-fMRI multi-site data can help to understand the inner working of the brain, the data acquisition and processing of this data has many challenges. One of the challenges is the variability of the data associated with different acquisitions sites, and different MRI machines vendors. Other factors such as population heterogeneity among different sites, with variables such as age and gender of the subjects, must also be considered. Given that most of the machine-learning models are developed using these rs-fMRI multi-site data sets, the intrinsic confounding effects can adversely affect the generalizability and reliability of these computational methods, as well as the imposition of upper limits on the classification scores. This work aims to identify the phenotypic and imaging variables producing the confounding effects, as well as to control these effects. Our goal is to maximize the classification scores obtained from the machine learning analysis of the Autism Brain Imaging Data Exchange (ABIDE) rs-fMRI multi-site data. To achieve this goal, we propose novel methods of stratification to produce homogeneous sub-samples of the 17 ABIDE sites, as well as the generation of new features from the static functional connectivity values, using multiple linear regression models, ComBat harmonization models, and normalization methods. The main results obtained with our statistical models and methods are an accuracy of 76.4%, sensitivity of 82.9%, and specificity of 77.0%, which are 8.8%, 20.5%, and 7.5% above the baseline classification scores obtained from the machine learning analysis of the static functional connectivity computed from the ABIDE rs-fMRI multi-site data.
PMID:37581850 | DOI:10.1007/s12021-023-09639-1



Estimated reading time: 5 minute(s)
Latest: Psychiatryai.com #RAISR4D Evidence

Cool Evidence: Engaging Young People and Students in Real-World Evidence

Real-Time Evidence Search [Psychiatry]

AI Research

Confounding Effects on the Performance of Machine Learning Analysis of Static Functional Connectivity Computed from rs-fMRI Multi-site Data
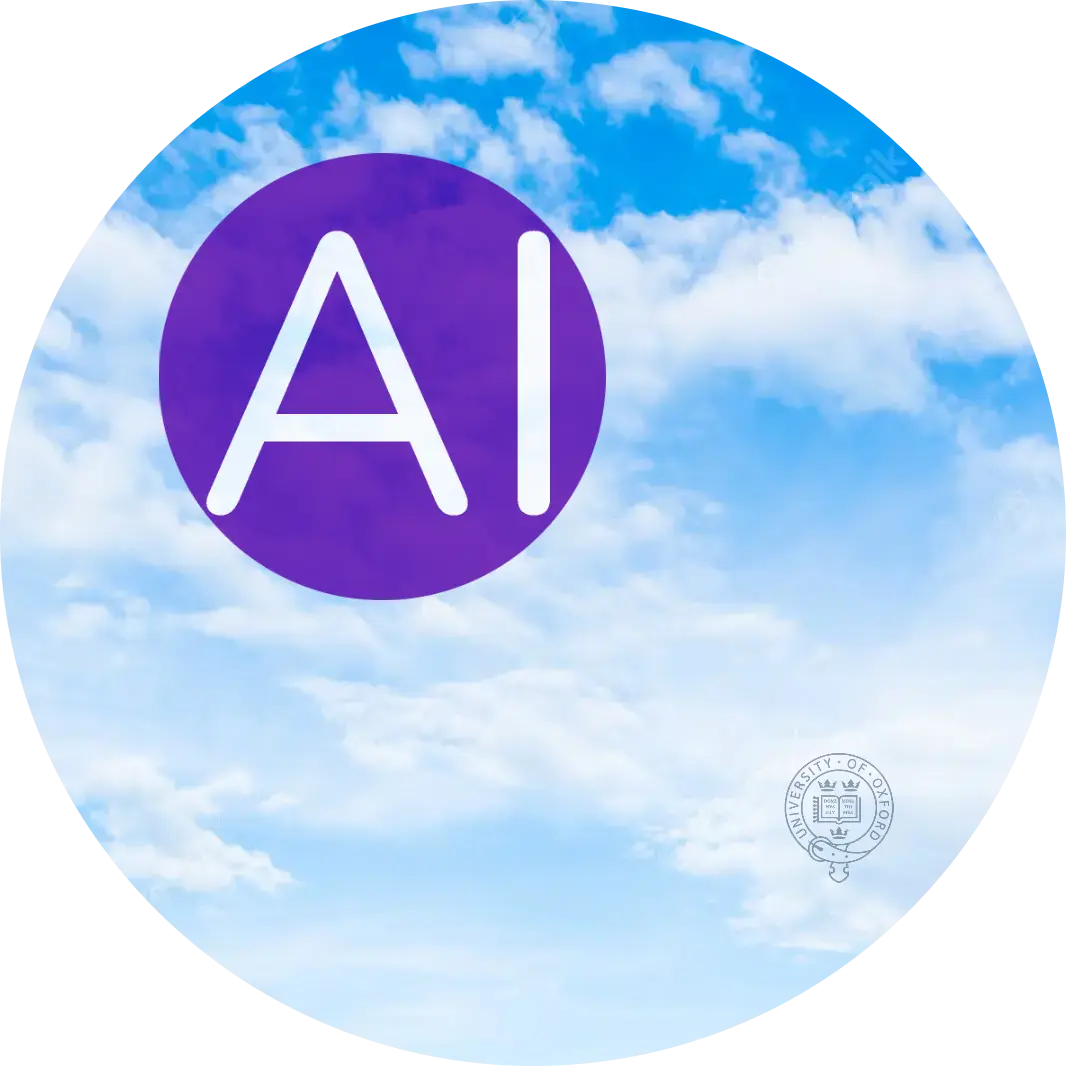
🌐 90 Days
Evidence Blueprint
Confounding Effects on the Performance of Machine Learning Analysis of Static Functional Connectivity Computed from rs-fMRI Multi-site Data
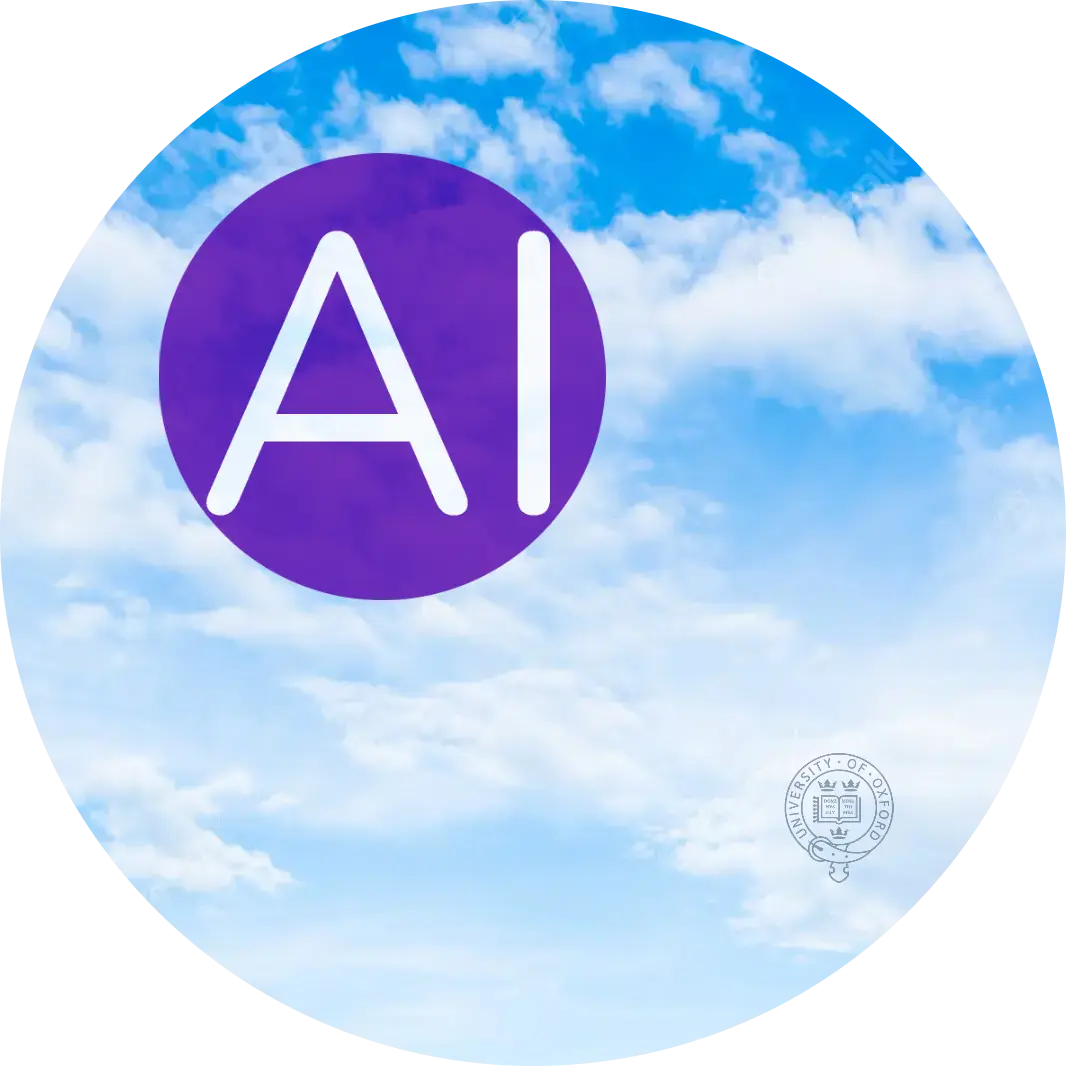
☊ AI-Driven Related Evidence Nodes
(recent articles with at least 5 words in title)
More Evidence
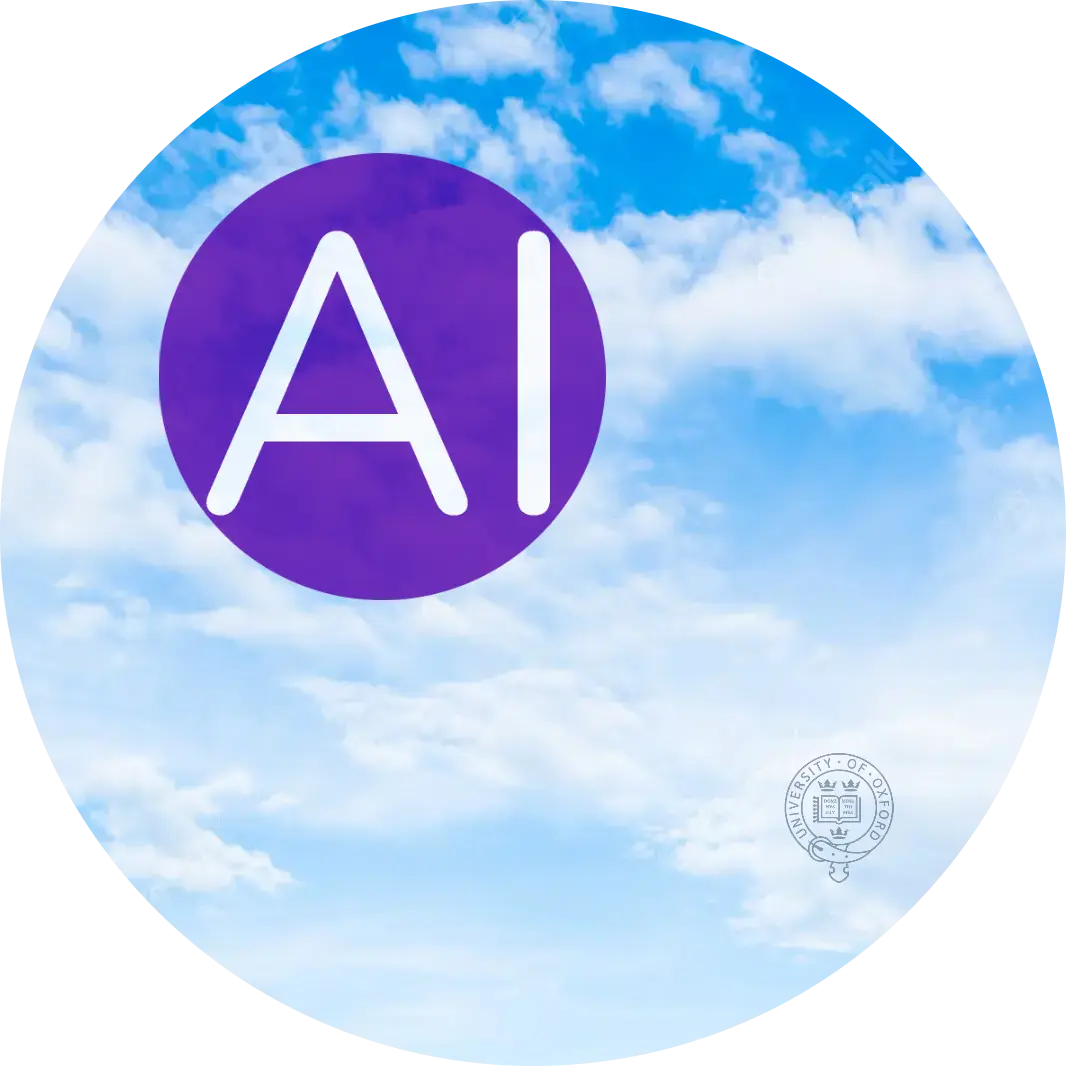